[ad_1]
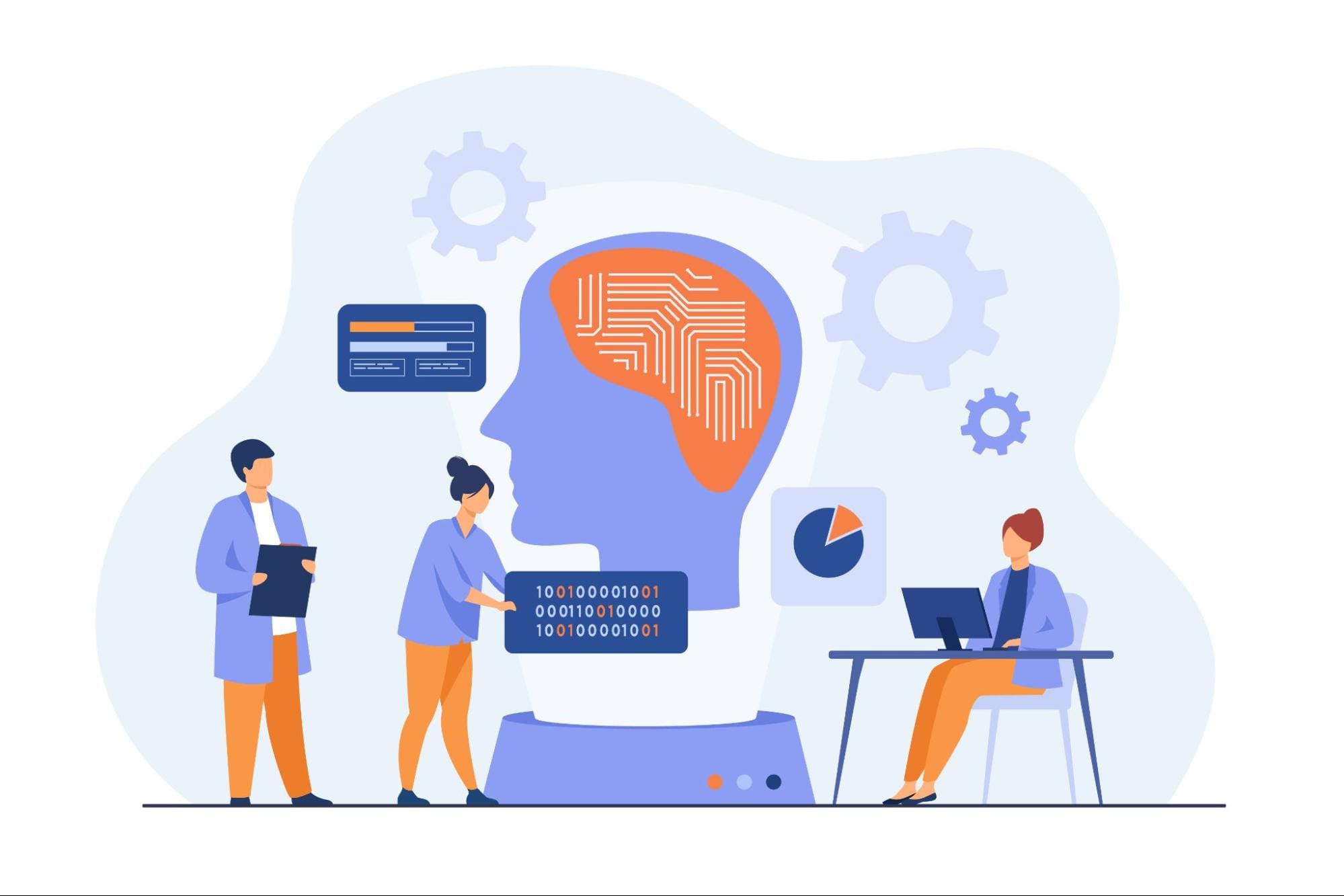
Picture by pch.vector on Freepik
Equipment Studying is a significant area with new investigation coming out commonly. It is a very hot discipline wherever academia and field preserve experimenting with new factors to make improvements to our day-to-day life.
In latest years, generative AI has been modifying the entire world thanks to the software of equipment mastering. For example, ChatGPT and Secure Diffusion. Even with 2023 dominated by generative AI, we need to be conscious of many more machine discovering breakthroughs.
In this article are the prime machine learning papers to examine in 2023 so you will not overlook the approaching tendencies.
1) Mastering the Elegance in Songs: Neural Singing Voice Beautifier
Singing Voice Beautifying (SVB) is a novel undertaking in generative AI that aims to enhance the newbie singing voice into a stunning just one. It’s particularly the analysis purpose of Liu et al. (2022) when they proposed a new generative design termed Neural Singing Voice Beautifier (NSVB).
The NSVB is a semi-supervised finding out design applying a latent-mapping algorithm that functions as a pitch corrector and increases vocal tone. The work guarantees to improve the musical field and is worth checking out.
2) Symbolic Discovery of Optimization Algorithms
Deep neural network models have come to be more substantial than ever, and a great deal exploration has been conducted to simplify the instruction approach. Modern investigate by the Google team (Chen et al. (2023)) has proposed a new optimization for the Neural Community named Lion (Developed Sign Momentum). The process displays that the algorithm is much more memory-productive and calls for a more compact learning charge than Adam. It’s great study that exhibits a lot of claims you should really not skip.
3) TimesNet: Temporal 2D-Variation Modeling for Common Time Sequence Examination
Time series assessment is a common use circumstance in a lot of enterprises For illustration, cost forecasting, anomaly detection, etc. On the other hand, there are many troubles to analyzing temporal knowledge only dependent on the latest information (1D data). That is why Wu et al. (2023) suggest a new technique identified as TimesNet to transform the 1D details into 2D info, which achieves terrific effectiveness in the experiment. You should read the paper to realize superior this new strategy as it would assist a lot long term time collection evaluation.
4) Choose: Open Pre-qualified Transformer Language Designs
Currently, we are in a generative AI period where by a lot of large language types were intensively created by organizations. Largely this sort of investigation would not launch their model or only be commercially readily available. Nevertheless, the Meta AI research group (Zhang et al. (2022)) attempts to do the opposite by publicly releasing the Open up Pre-skilled Transformers (Decide) design that could be comparable with the GPT-3. The paper is a terrific start off to comprehension the Opt product and the investigate depth, as the group logs all the detail in the paper.
5) REaLTabFormer: Generating Realistic Relational and Tabular Info using Transformers
The generative design is not constrained to only generating text or shots but also tabular knowledge. This produced facts is frequently termed artificial facts. Lots of designs had been produced to deliver synthetic tabular details, but pretty much no design to generate relational tabular synthetic knowledge. This is particularly the goal of Solatorio and Dupriez (2023) investigation generating a design named REaLTabFormer for synthetic relational knowledge. The experiment has revealed that the consequence is accurately near to the existing synthetic product, which could be prolonged to numerous applications.
6) Is Reinforcement Understanding (Not) for Pure Language Processing?: Benchmarks, Baselines, and Setting up Blocks for Pure Language Coverage Optimization
Reinforcement Studying conceptually is an outstanding option for the Organic Language Processing activity, but is it real? This is a dilemma that Ramamurthy et al. (2022) consider to response. The researcher introduces many library and algorithm that exhibits where Reinforcement Mastering methods have an edge compared to the supervised strategy in the NLP responsibilities. It is a advised paper to go through if you want an substitute for your skillset.
7) Tune-A-Video: A person-Shot Tuning of Impression Diffusion Models for Textual content-to-Online video Generation
Text-to-impression technology was massive in 2022, and 2023 would be projected on text-to-video (T2V) functionality. Investigation by Wu et al. (2022) exhibits how T2V can be extended on quite a few methods. The exploration proposes a new Tune-a-Video clip method that supports T2V tasks these as issue and object alter, design transfer, attribute editing, and so forth. It’s a terrific paper to read through if you are fascinated in text-to-video clip investigation.
8) PyGlove: Successfully Exchanging ML Tips as Code
Successful collaboration is the important to achievement on any workforce, specially with the growing complexity within equipment learning fields. To nurture performance, Peng et al. (2023) existing a PyGlove library to share ML thoughts conveniently. The PyGlove strategy is to capture the procedure of ML study through a checklist of patching procedures. The list can then be reused in any experiments scene, which increases the team’s performance. It is research that tries to clear up a machine studying issue that several have not carried out however, so it is worth studying.
8) How Near is ChatGPT to Human Professionals? Comparison Corpus, Analysis, and Detection
ChatGPT has modified the earth so substantially. It’s risk-free to say that the pattern would go upward from below as the general public is by now in favor of making use of ChatGPT. Nonetheless, how is the ChatGPT latest end result in contrast to the Human Specialists? It’s just a problem that Guo et al. (2023) try out to solution. The group experimented with to gather facts from professionals and ChatGPT prompt success, which they in contrast. The consequence displays that implicit dissimilarities amongst ChatGPT and experts ended up there. The research is a little something that I come to feel would be retained questioned in the future as the generative AI design would hold rising in excess of time, so it’s really worth reading.
2023 is a excellent year for device discovering investigate revealed by the current trend, specially generative AI this kind of as ChatGPT and Steady Diffusion. There is much promising investigation that I experience we ought to not skip since it is shown promising benefits that might improve the existing typical. In this post, I have proven you 9 prime ML papers to read, ranging from the generative design, time collection model to workflow performance. I hope it helps.
Cornellius Yudha Wijaya is a facts science assistant manager and info writer. While doing the job comprehensive-time at Allianz Indonesia, he loves to share Python and Knowledge ideas through social media and writing media.
[ad_2]
Resource backlink