[ad_1]
To coach brokers to interact properly with individuals, we need to be in a position to measure development. But human interaction is advanced and measuring progress is complicated. In this function we designed a approach, termed the Standardised Check Suite (STS), for evaluating brokers in temporally extended, multi-modal interactions. We examined interactions that consist of human individuals asking brokers to carry out duties and reply questions in a 3D simulated setting.
The STS methodology sites brokers in a established of behavioural eventualities mined from true human conversation information. Brokers see a replayed circumstance context, acquire an instruction, and are then given control to entire the conversation offline. These agent continuations are recorded and then sent to human raters to annotate as good results or failure. Brokers are then rated in accordance to the proportion of situations on which they do well.
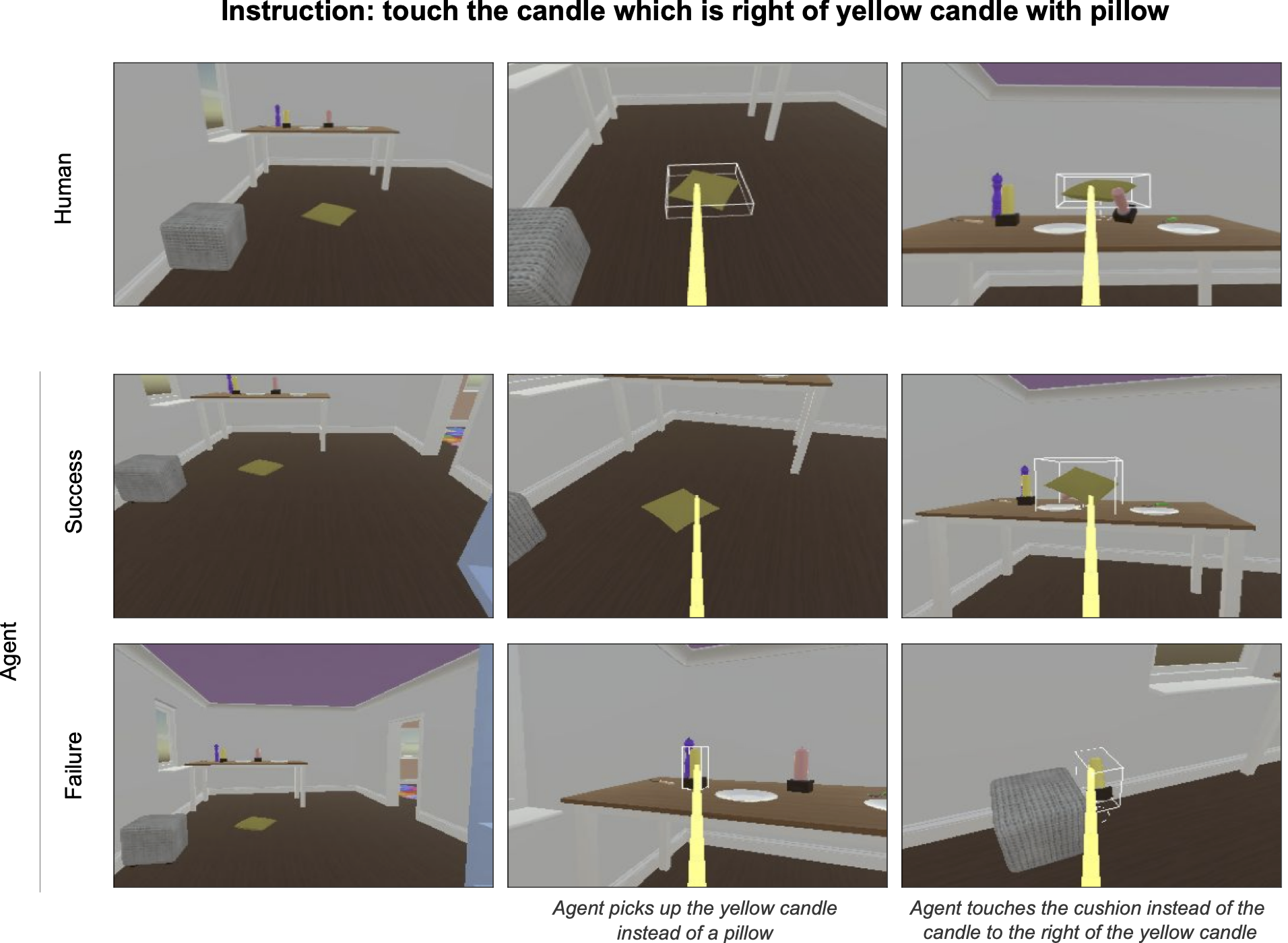
Quite a few of the behaviours that are next mother nature to people in our day-to-working day interactions are complicated to place into words and phrases, and not possible to formalise. So, the system relied on for fixing online games (like Atari, Go, DotA, and Starcraft) with reinforcement learning is not going to function when we check out to instruct brokers to have fluid and productive interactions with humans. For illustration, feel about the variance amongst these two concerns: “Who won this game of Go?” vs . “What are you looking at?” In the initially situation, we can compose a piece of pc code that counts the stones on the board at the conclude of the recreation and decides the winner with certainty. In the next situation, we have no notion how to codify this: the solution may possibly depend on the speakers, the dimension and shapes of the objects associated, regardless of whether the speaker is joking, and other facets of the context in which the utterance is offered. Human beings intuitively fully grasp the myriad of relevant elements involved in answering this seemingly mundane query.
Interactive analysis by human members can serve as a touchstone for comprehending agent overall performance, but this is noisy and pricey. It is challenging to management the precise recommendations that people give to brokers when interacting with them for evaluation. This kind of analysis is also in serious-time, so it is much too gradual to depend on for swift progress. Previous is effective have relied on proxies to interactive evaluation. Proxies, this sort of as losses and scripted probe responsibilities (e.g. “lift the x” the place x is randomly chosen from the surroundings and the results function is painstakingly hand-crafted), are handy for getting perception into agents promptly, but do not truly correlate that well with interactive analysis. Our new method has pros, mostly affording regulate and speed to a metric that intently aligns with our top intention – to generate agents that interact well with individuals.
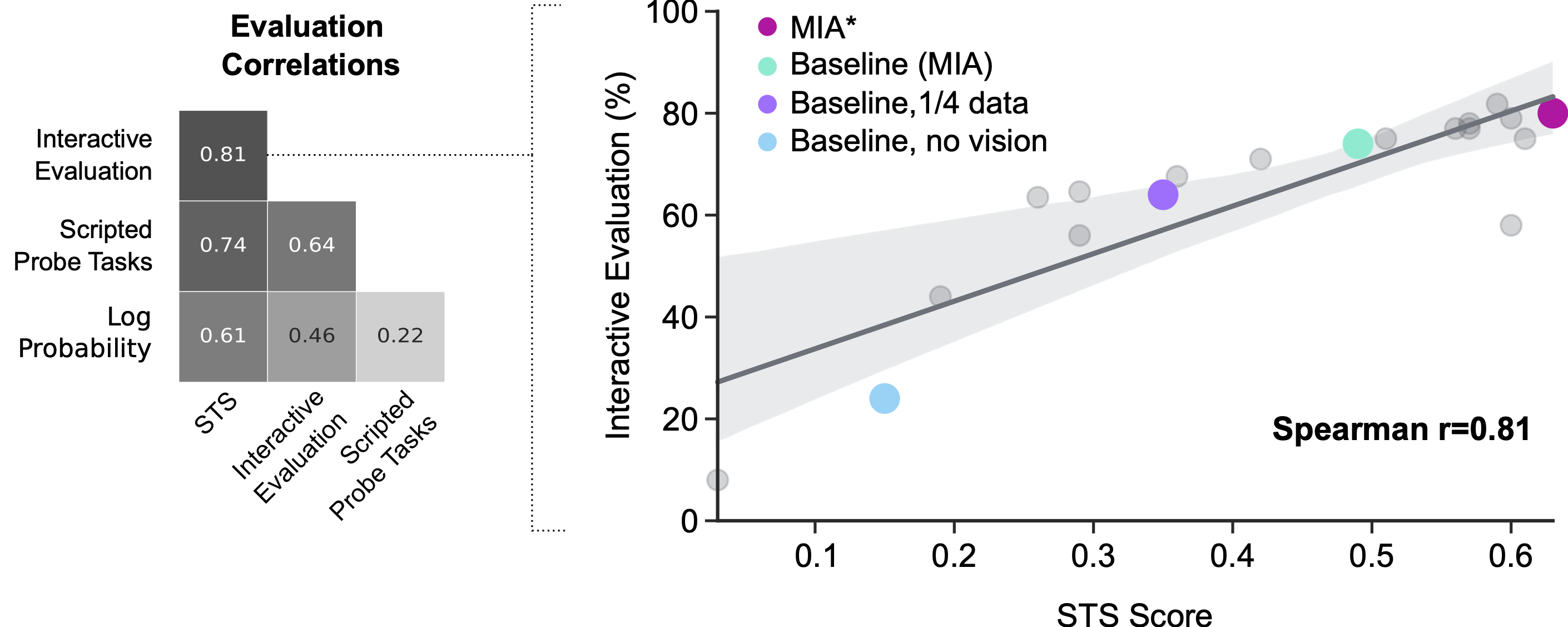
The enhancement of MNIST, ImageNet and other human-annotated datasets has been necessary for development in equipment understanding. These datasets have authorized researchers to teach and appraise classification products for a a person-time charge of human inputs. The STS methodology aims to do the identical for human-agent interaction analysis. This analysis technique nonetheless necessitates individuals to annotate agent continuations even so, early experiments counsel that automation of these annotations may be attainable, which would permit rapidly and effective automated evaluation of interactive agents. In the meantime, we hope that other researchers can use the methodology and procedure style and design to accelerate their personal analysis in this spot.
[ad_2]
Supply website link