[ad_1]
Individuals are an interactive species. We interact with the bodily entire world and with just one one more. For synthetic intelligence (AI) to be commonly helpful, it ought to be equipped to interact capably with human beings and their atmosphere. In this work we current the Multimodal Interactive Agent (MIA), which blends visual perception, language comprehension and manufacturing, navigation, and manipulation to interact in prolonged and often astonishing actual physical and linguistic interactions with people.
We construct upon the strategy introduced by Abramson et al. (2020), which largely uses imitation discovering to prepare brokers. Immediately after training, MIA shows some rudimentary clever behaviour that we hope to afterwards refine employing human opinions. This get the job done focuses on the creation of this smart behavioural prior, and we leave even more suggestions-primarily based discovering for foreseeable future get the job done.
We established the Playhouse environment, a 3D digital surroundings composed of a randomised set of rooms and a significant variety of domestic interactable objects, to give a room and location for humans and agents to interact collectively. Human beings and agents can interact in the Playhouse by managing virtual robots that locomote, manipulate objects, and converse by way of text. This virtual natural environment permits a vast array of positioned dialogues, ranging from uncomplicated recommendations (e.g., “Please select up the book from the floor and position it on the blue bookshelf”) to creative enjoy (e.g., “Bring foods to the desk so that we can eat”).
We collected human illustrations of Playhouse interactions applying language online games, a assortment of cues prompting humans to improvise specified behaviours. In a language recreation just one participant (the setter) receives a prewritten prompt indicating a kind of endeavor to suggest to the other player (the solver). For illustration, the setter could get the prompt “Ask the other participant a issue about the existence of an item,” and after some exploration, the setter could question, ”Please tell me no matter if there is a blue duck in a home that does not also have any home furnishings.” To make sure ample behavioural variety, we also provided totally free-type prompts, which granted setters absolutely free preference to improvise interactions (E.g. “Now consider any item that you like and strike the tennis ball off the stool so that it rolls around the clock, or someplace in the vicinity of it.”). In full, we collected 2.94 decades of authentic-time human interactions in the Playhouse.
.jpg)
Our coaching strategy is a blend of supervised prediction of human actions (behavioural cloning) and self-supervised mastering. When predicting human steps, we observed that utilizing a hierarchical manage method significantly enhanced agent overall performance. In this placing, the agent receives new observations roughly 4 instances per second. For each and every observation, it produces a sequence of open-loop motion actions and optionally emits a sequence of language actions. In addition to behavioural cloning we use a sort of self-supervised learning, which jobs brokers with classifying no matter whether certain eyesight and language inputs belong to the exact or various episodes.
To appraise agent functionality, we questioned human members to interact with brokers and give binary feed-back indicating no matter whether the agent efficiently carried out an instruction. MIA achieves in excess of 70% achievements price in human-rated online interactions, symbolizing 75% of the achievements level that humans on their own realize when they play as solvers. To far better fully grasp the part of numerous parts in MIA, we executed a sequence of ablations, eradicating, for instance, visible or language inputs, the self-supervised loss, or the hierarchical management.
Present-day device understanding study has uncovered impressive regularities of overall performance with regard to distinct scale parameters in particular, design effectiveness scales as a energy-law with dataset sizing, product dimensions, and compute. These effects have been most crisply noted in the language domain, which is characterised by substantial dataset sizes and very evolved architectures and teaching protocols. In this perform, nevertheless, we are in a decidedly diverse regime – with comparatively compact datasets and multimodal, multi-activity aim capabilities instruction heterogeneous architectures. However, we demonstrate apparent consequences of scaling: as we increase dataset and product measurement, performance increases appreciably.
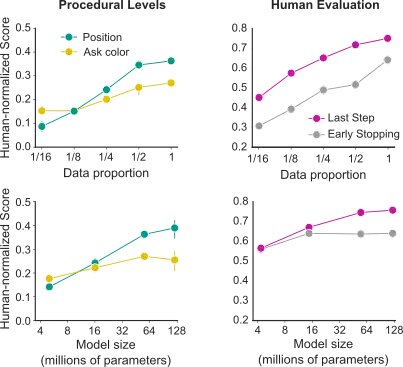
In an best case, teaching becomes far more efficient specified a reasonably massive dataset, as information is transferred concerning activities. To look into how best our situation are, we examined how much details is essential to discover to interact with a new, formerly unseen object and to master how to follow a new, formerly unheard command / verb. We partitioned our info into background facts and data involving a language instruction referring to the item or the verb. When we reintroduced the knowledge referring to the new item, we uncovered that much less than 12 hrs of human conversation was more than enough to obtain the ceiling efficiency. Analogously, when we launched the new command or verb ‘to clear’ (i.e. to take out all objects from a surface area), we identified that only 1 hour of human demonstrations was enough to access ceiling overall performance in responsibilities involving this term.
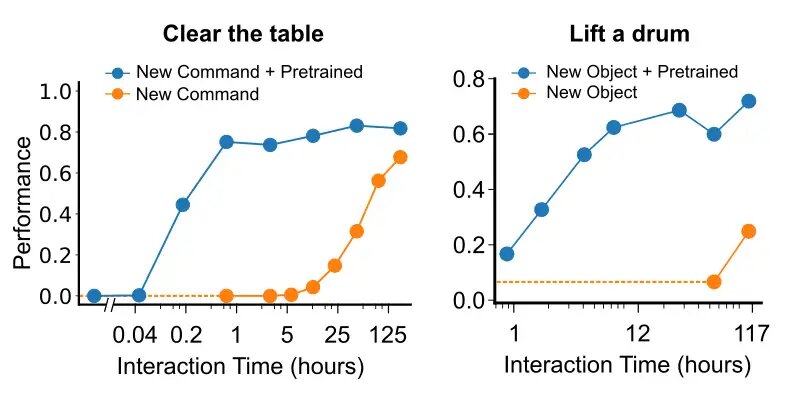
MIA displays startlingly wealthy conduct, together with a variety of behaviours that were being not preconceived by researchers, together with tidying a area, discovering several specified objects, and asking clarifying thoughts when an instruction is ambiguous. These interactions continuously encourage us. Nonetheless, the open-endedness of MIA’s behaviour provides enormous issues for quantitative analysis. Establishing thorough methodologies to seize and analyse open up-ended behaviour in human-agent interactions will be an crucial aim in our long term operate.
For a extra thorough description of our get the job done, see our paper.
[ad_2]
Supply connection