[ad_1]
Reward is the driving pressure for reinforcement studying (RL) agents. Given its central purpose in RL, reward is typically assumed to be suitably common in its expressivity, as summarized by Sutton and Littman’s reward hypothesis:
“…all of what we necessarily mean by plans and purposes can be well considered of as maximization of the envisioned price of the cumulative sum of a gained scalar signal (reward).”
– SUTTON (2004), LITTMAN (2017)
In our get the job done, we get to start with ways toward a systematic examine of this speculation. To do so, we take into consideration the pursuing considered experiment involving Alice, a designer, and Bob, a discovering agent:
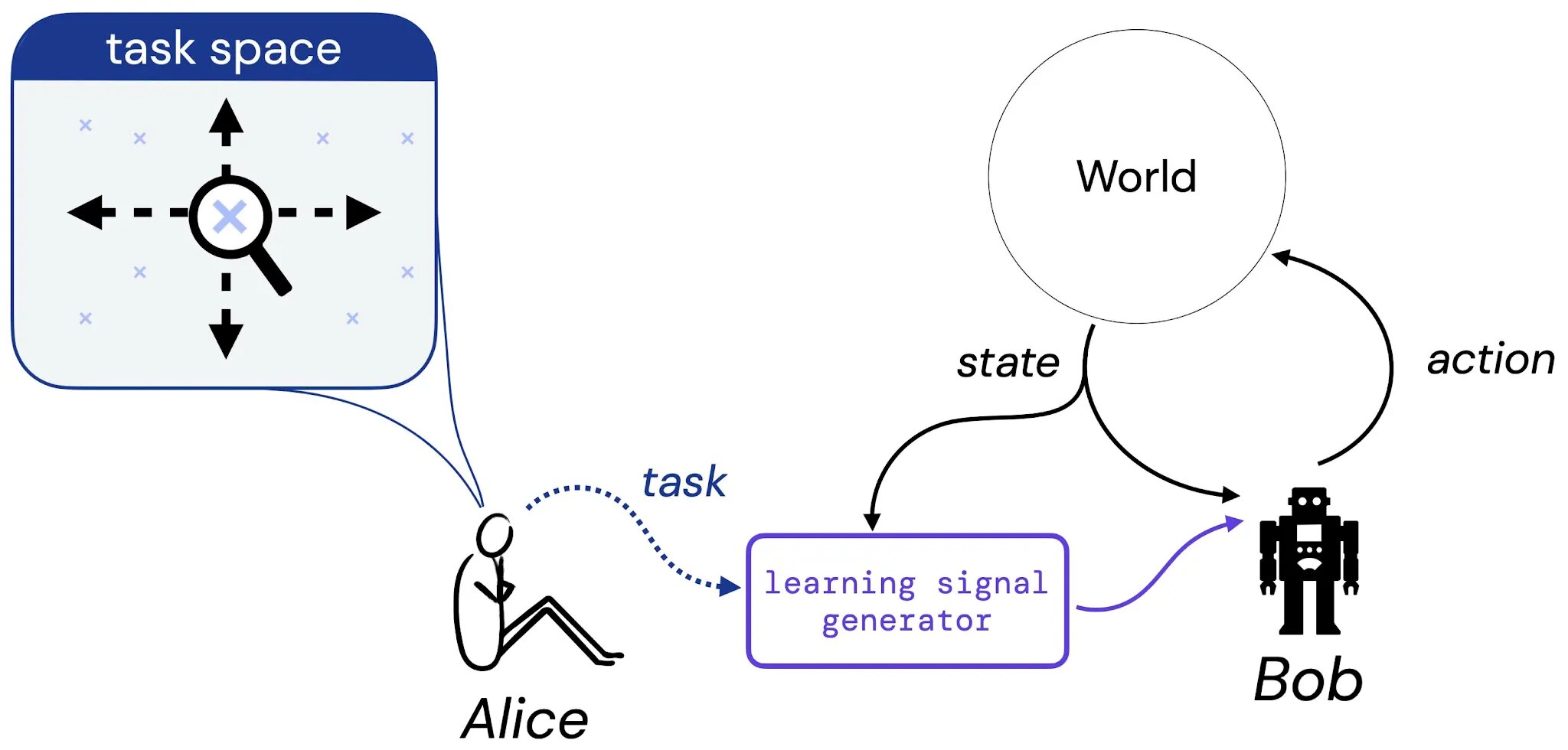
We suppose that Alice thinks of a activity she may possibly like Bob to study to address – this task could be in the kind a a all-natural language description (“balance this pole”), an imagined point out of affairs (“reach any of the profitable configurations of a chess board”), or something additional standard like a reward or value purpose. Then, we imagine Alice translates her decision of activity into some generator that will supply understanding signal (these as reward) to Bob (a discovering agent), who will study from this signal throughout his lifetime. We then floor our examine of the reward hypothesis by addressing the adhering to question: presented Alice’s preference of job, is there generally a reward operate that can express this task to Bob?
What is a task?
To make our study of this concern concrete, we initial limit target to three sorts of process. In particular, we introduce three undertaking kinds that we think seize sensible kinds of responsibilities: 1) A established of appropriate procedures (Soap), 2) A coverage purchase (PO), and 3) A trajectory order (TO). These three forms of duties symbolize concrete cases of the varieties of task we may want an agent to discover to fix.
.jpg)
We then study regardless of whether reward is capable of capturing each individual of these process styles in finite environments. Crucially, we only focus awareness on Markov reward features for instance, offered a state area that is sufficient to kind a task this kind of as (x,y) pairs in a grid environment, is there a reward perform that only is dependent on this same point out space that can capture the undertaking?
Initially Major Consequence
Our first key consequence exhibits that for each individual of the a few endeavor types, there are environment-task pairs for which there is no Markov reward perform that can seize the job. A person case in point of these a pair is the “go all the way all-around the grid clockwise or counterclockwise” task in a common grid earth:
.jpg)
This process is by natural means captured by a Soap that is made up of two appropriate policies: the “clockwise” policy (in blue) and the “counterclockwise” plan (in purple). For a Markov reward function to categorical this process, it would need to have to make these two guidelines strictly bigger in value than all other deterministic procedures. Having said that, there is no these types of Markov reward perform: the optimality of a solitary “move clockwise” action will rely on whether the agent was already going in that way in the earlier. Given that the reward function should be Markov, it can’t convey this variety of data. Identical illustrations display that Markov reward are unable to capture every single plan buy and trajectory purchase, way too.
Second Major Final result
Given that some responsibilities can be captured and some can’t, we following take a look at no matter if there is an effective treatment for figuring out irrespective of whether a specified undertaking can be captured by reward in a specified ecosystem. Even more, if there is a reward functionality that captures the supplied task, we would preferably like to be equipped to output this kind of a reward purpose. Our next consequence is a favourable result which says that for any finite environment-process pair, there is a course of action that can 1) decide regardless of whether the activity can be captured by Markov reward in the provided surroundings, and 2) outputs the ideal reward purpose that just conveys the process, when these types of a function exists.
This operate establishes preliminary pathways toward comprehending the scope of the reward hypothesis, but there is significantly still to be carried out to generalize these final results past finite environments, Markov rewards, and uncomplicated notions of “task” and “expressivity”. We hope this function provides new conceptual views on reward and its put in reinforcement learning.
[ad_2]
Supply url