[ad_1]
To study ocean currents, researchers release GPS-tagged buoys in the ocean and record their velocities to reconstruct the currents that transport them. These buoy details are also applied to detect “divergences,” which are spots the place h2o rises up from down below the surface or sinks beneath it.
By accurately predicting currents and pinpointing divergences, experts can much more specifically forecast the climate, approximate how oil will distribute right after a spill, or evaluate strength transfer in the ocean. A new design that incorporates machine discovering can make much more correct predictions than typical styles do, a new review experiences.
A multidisciplinary analysis crew which includes computer system researchers at MIT and oceanographers has identified that a common statistical model commonly used on buoy information can struggle to accurately reconstruct currents or establish divergences because it can make unrealistic assumptions about the behavior of water.
The researchers designed a new product that incorporates understanding from fluid dynamics to improved replicate the physics at do the job in ocean currents. They show that their method, which only necessitates a little quantity of further computational cost, is more correct at predicting currents and identifying divergences than the regular model.
This new model could assist oceanographers make extra accurate estimates from buoy facts, which would enable them to much more successfully keep track of the transportation of biomass (these as Sargassum seaweed), carbon, plastics, oil, and nutrients in the ocean. This information is also important for comprehending and tracking local weather improve.
“Our method captures the actual physical assumptions far more appropriately and a lot more accurately. In this scenario, we know a great deal of the physics currently. We are offering the product a minimal little bit of that information so it can concentrate on mastering the things that are critical to us, like what are the currents absent from the buoys, or what is this divergence and in which is it happening?” says senior creator Tamara Broderick, an affiliate professor in MIT’s Office of Electrical Engineering and Personal computer Science (EECS) and a member of the Laboratory for Information and Determination Units and the Institute for Details, Techniques, and Modern society.
Broderick’s co-authors consist of guide creator Renato Berlinghieri, an electrical engineering and laptop science graduate university student Brian L. Trippe, a postdoc at Columbia College David R. Burt and Ryan Giordano, MIT postdocs Kaushik Srinivasan, an assistant researcher in atmospheric and ocean sciences at the University of California at Los Angeles Tamay Özgökmen, professor in the Division of Ocean Sciences at the College of Miami and Junfei Xia, a graduate college student at the College of Miami. The study will be offered at the Intercontinental Meeting on Machine Learning.
Diving into the knowledge
Oceanographers use facts on buoy velocity to forecast ocean currents and establish “divergences” exactly where drinking water rises to the area or sinks deeper.
To estimate currents and obtain divergences, oceanographers have used a machine-learning strategy known as a Gaussian procedure, which can make predictions even when facts are sparse. To do the job effectively in this case, the Gaussian method will have to make assumptions about the info to create a prediction.
A common way of making use of a Gaussian method to oceans knowledge assumes the latitude and longitude parts of the latest are unrelated. But this assumption isn’t physically correct. For occasion, this existing product indicates that a current’s divergence and its vorticity (a whirling movement of fluid) operate on the same magnitude and duration scales. Ocean experts know this is not real, Broderick suggests. The earlier design also assumes the frame of reference issues, which signifies fluid would behave in another way in the latitude compared to the longitude route.
“We have been pondering we could handle these complications with a design that incorporates the physics,” she states.
They constructed a new model that makes use of what is identified as a Helmholtz decomposition to correctly depict the principles of fluid dynamics. This strategy types an ocean existing by breaking it down into a vorticity ingredient (which captures the whirling movement) and a divergence element (which captures h2o soaring or sinking).
In this way, they give the design some primary physics understanding that it makes use of to make additional accurate predictions.
This new product makes use of the exact same data as the aged design. And whilst their technique can be a lot more computationally intense, the researchers exhibit that the supplemental cost is comparatively little.
Buoyant overall performance
They evaluated the new design utilizing synthetic and real ocean buoy info. Mainly because the artificial info were fabricated by the researchers, they could review the model’s predictions to ground-real truth currents and divergences. But simulation involves assumptions that may perhaps not replicate true life, so the researchers also tested their product using details captured by authentic buoys released in the Gulf of Mexico.
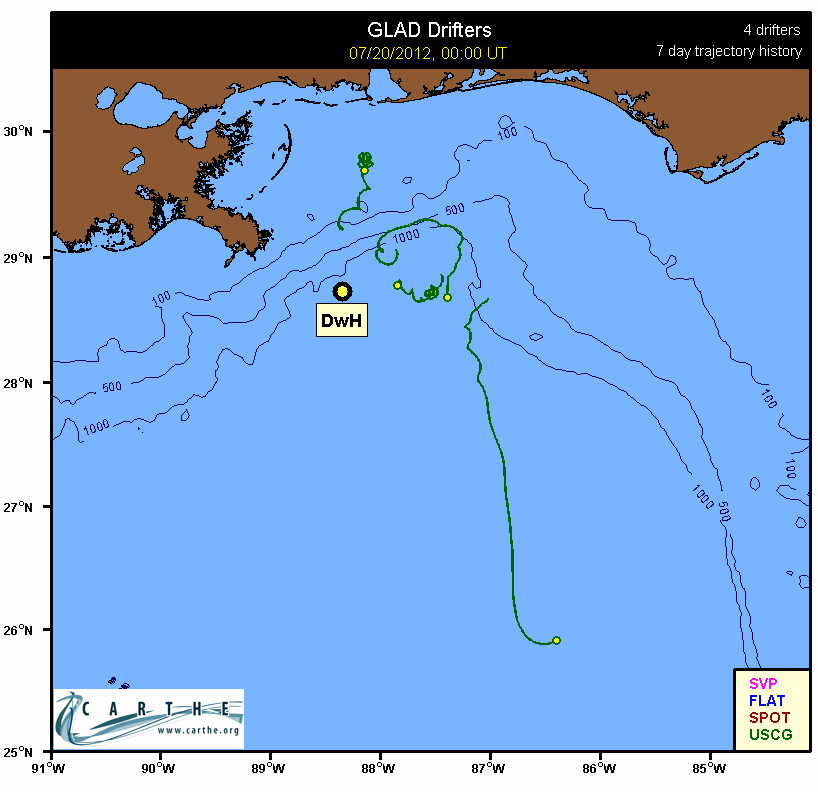
Credit history: Consortium of State-of-the-art Exploration for Transportation of Hydrocarbons in the Surroundings
In each individual scenario, their method shown outstanding functionality for both tasks, predicting currents and pinpointing divergences, when in comparison to the regular Gaussian method and an additional device-mastering technique that used a neural network. For case in point, in a person simulation that integrated a vortex adjacent to an ocean latest, the new technique properly predicted no divergence whilst the preceding Gaussian process technique and the neural community approach each predicted a divergence with incredibly superior self confidence.
The approach is also good at identifying vortices from a compact set of buoys, Broderick provides.
Now that they have demonstrated the efficiency of applying a Helmholtz decomposition, the researchers want to incorporate a time element into their design, given that currents can range about time as nicely as space. In addition, they want to superior capture how sound impacts the data, these kinds of as winds that occasionally influence buoy velocity. Separating that sound from the information could make their technique much more exact.
“Our hope is to take this noisily noticed industry of velocities from the buoys, and then say what is the genuine divergence and precise vorticity, and predict absent from those buoys, and we feel that our new strategy will be handy for this,” she states.
“The authors cleverly combine recognised behaviors from fluid dynamics to product ocean currents in a adaptable design,” suggests Massimiliano Russo, an associate biostatistician at Brigham and Women’s Medical center and instructor at Harvard Professional medical Faculty, who was not included with this function. “The resulting method retains the adaptability to design the nonlinearity in the currents but can also characterize phenomena this sort of as vortices and connected currents that would only be discovered if the fluid dynamic composition is integrated into the model. This is an outstanding case in point of exactly where a adaptable model can be significantly enhanced with a very well thought and scientifically sound specification.”
This study is supported by the Office of Naval Investigation as a result of a Multi College Analysis Initiative (MURI) application titled “Equipment Mastering for Submesoscale Characterization, Ocean Prediction, and Exploration (ML-SCOPE).” It is also supported in part by a National Science Foundation (NSF) Career Award and the Rosenstiel Faculty of Maritime, Atmospheric, and Earth Science at the College of Miami.
[ad_2]
Resource url