[ad_1]
In the movie “Top Gun: Maverick,” Maverick, performed by Tom Cruise, is charged with instruction young pilots to comprehensive a seemingly difficult mission — to fly their jets deep into a rocky canyon, staying so minimal to the floor they are unable to be detected by radar, then quickly climb out of the canyon at an serious angle, averting the rock partitions. Spoiler alert: With Maverick’s enable, these human pilots accomplish their mission.
A machine, on the other hand, would struggle to finish the exact pulse-pounding activity. To an autonomous plane, for instance, the most straightforward path toward the focus on is in conflict with what the device wants to do to avoid colliding with the canyon partitions or keeping undetected. Lots of present AI approaches are not in a position to prevail over this conflict, acknowledged as the stabilize-stay away from difficulty, and would be not able to achieve their aim safely and securely.
MIT researchers have created a new approach that can clear up sophisticated stabilize-stay away from complications far better than other techniques. Their device-mastering strategy matches or exceeds the protection of existing strategies while furnishing a tenfold improve in stability, meaning the agent reaches and remains stable within just its purpose region.
In an experiment that would make Maverick proud, their approach correctly piloted a simulated jet aircraft through a narrow corridor with out crashing into the ground.
“This has been a longstanding, complicated dilemma. A ton of people today have seemed at it but didn’t know how to handle this sort of higher-dimensional and complicated dynamics,” suggests Chuchu Lover, the Wilson Assistant Professor of Aeronautics and Astronautics, a member of the Laboratory for Facts and Final decision Units (LIDS), and senior creator of a new paper on this technique.
Supporter is joined by guide creator Oswin So, a graduate student. The paper will be introduced at the Robotics: Science and Methods conference.
The stabilize-steer clear of challenge
A lot of approaches deal with complicated stabilize-stay away from challenges by simplifying the technique so they can solve it with simple math, but the simplified results often do not keep up to serious-planet dynamics.
Far more productive strategies use reinforcement studying, a equipment-studying technique in which an agent learns by trial-and-mistake with a reward for behavior that gets it nearer to a intention. But there are genuinely two objectives in this article — remain steady and stay clear of road blocks — and obtaining the correct equilibrium is tedious.
The MIT scientists broke the challenge down into two actions. To start with, they reframe the stabilize-steer clear of issue as a constrained optimization challenge. In this set up, solving the optimization allows the agent to achieve and stabilize to its intention, this means it stays inside of a particular region. By applying constraints, they ensure the agent avoids road blocks, So clarifies.
Then for the 2nd action, they reformulate that constrained optimization issue into a mathematical representation recognized as the epigraph sort and fix it using a deep reinforcement learning algorithm. The epigraph kind allows them bypass the issues other approaches face when applying reinforcement discovering.
“But deep reinforcement understanding isn’t developed to remedy the epigraph variety of an optimization challenge, so we couldn’t just plug it into our issue. We had to derive the mathematical expressions that perform for our program. As soon as we experienced those people new derivations, we combined them with some present engineering tricks utilized by other approaches,” So says.
No details for second location
To take a look at their solution, they made a number of management experiments with various initial disorders. For occasion, in some simulations, the autonomous agent demands to attain and stay inside a objective area although building drastic maneuvers to keep away from road blocks that are on a collision class with it.
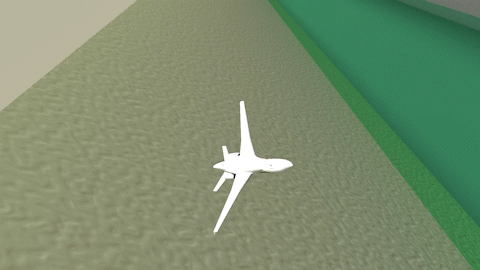
Courtesy of the researchers
When as opposed with quite a few baselines, their strategy was the only a person that could stabilize all trajectories though sustaining security. To push their strategy even further more, they applied it to fly a simulated jet aircraft in a state of affairs one particular could see in a “Top Gun” movie. The jet experienced to stabilize to a focus on close to the floor even though maintaining a incredibly minimal altitude and keeping inside a slim flight corridor.
This simulated jet design was open up-sourced in 2018 and had been made by flight management professionals as a testing obstacle. Could researchers produce a state of affairs that their controller could not fly? But the design was so complex it was hard to operate with, and it even now couldn’t deal with complicated scenarios, Enthusiast says.
The MIT researchers’ controller was able to stop the jet from crashing or stalling even though stabilizing to the intention significantly much better than any of the baselines.
In the long term, this method could be a beginning stage for coming up with controllers for hugely dynamic robots that must fulfill safety and stability demands, like autonomous delivery drones. Or it could be implemented as element of greater system. Possibly the algorithm is only activated when a car skids on a snowy highway to assist the driver securely navigate back again to a secure trajectory.
Navigating excessive eventualities that a human would not be equipped to cope with is exactly where their solution seriously shines, So provides.
“We believe that that a goal we need to attempt for as a industry is to give reinforcement studying the protection and security guarantees that we will have to have to offer us with assurance when we deploy these controllers on mission-essential devices. We believe this is a promising to start with step towards reaching that intention,” he states.
Transferring ahead, the researchers want to boost their procedure so it is superior capable to take uncertainty into account when solving the optimization. They also want to investigate how nicely the algorithm performs when deployed on hardware, given that there will be mismatches in between the dynamics of the product and these in the serious world.
“Professor Fan’s group has enhanced reinforcement mastering performance for dynamical methods in which security issues. Instead of just hitting a goal, they create controllers that make sure the method can reach its target safely and securely and remain there indefinitely,” claims Stanley Bak, an assistant professor in the Division of Pc Science at Stony Brook University, who was not concerned with this exploration. “Their enhanced formulation lets the productive era of safe controllers for sophisticated scenarios, such as a 17-state nonlinear jet plane model developed in aspect by scientists from the Air Power Investigation Lab (AFRL), which incorporates nonlinear differential equations with raise and drag tables.”
The operate is funded, in section, by MIT Lincoln Laboratory underneath the Safety in Aerobatic Flight Regimes application.
[ad_2]
Supply website link