[ad_1]
Efficiently managing the nuclear fusion plasma in a tokamak with deep reinforcement finding out
To fix the worldwide electrical power disaster, scientists have long sought a supply of cleanse, limitless power. Nuclear fusion, the response that powers the stars of the universe, is 1 contender. By smashing and fusing hydrogen, a widespread ingredient of seawater, the highly effective process releases big quantities of strength. Listed here on earth, a single way researchers have recreated these extraordinary disorders is by employing a tokamak, a doughnut-formed vacuum surrounded by magnetic coils, that is employed to include a plasma of hydrogen that is hotter than the main of the Sunlight. On the other hand, the plasmas in these equipment are inherently unstable, generating sustaining the procedure demanded for nuclear fusion a complex problem. For instance, a management process wants to coordinate the tokamak’s lots of magnetic coils and modify the voltage on them thousands of periods per second to make sure the plasma by no means touches the walls of the vessel, which would final result in warmth reduction and possibly problems. To assist resolve this difficulty and as part of DeepMind’s mission to progress science, we collaborated with the Swiss Plasma Center at EPFL to acquire the initially deep reinforcement mastering (RL) program to autonomously find how to management these coils and productively consist of the plasma in a tokamak, opening new avenues to progress nuclear fusion study.
In a paper printed now in Mother nature, we describe how we can correctly manage nuclear fusion plasma by building and operating controllers on the Variable Configuration Tokamak (TCV) in Lausanne, Switzerland. Using a learning architecture that combines deep RL and a simulated natural environment, we manufactured controllers that can the two retain the plasma continual and be utilised to accurately sculpt it into various designs. This “plasma sculpting” shows the RL method has properly managed the superheated make a difference and – importantly – allows experts to examine how the plasma reacts under different circumstances, bettering our understanding of fusion reactors.
“In the past two several years DeepMind has shown AI’s opportunity to accelerate scientific progress and unlock entirely new avenues of exploration throughout biology, chemistry, arithmetic and now physics.”
Demis Hassabis, Co-founder and CEO, DeepMind
This work is yet another strong case in point of how equipment understanding and skilled communities can appear alongside one another to tackle grand worries and accelerate scientific discovery. Our crew is tricky at do the job implementing this tactic to fields as assorted as quantum chemistry, pure arithmetic, product design, weather forecasting, and additional, to fix elementary problems and make certain AI gains humanity.
Studying when information is really hard to acquire
Study into nuclear fusion is currently minimal by researchers’ capacity to run experiments. Although there are dozens of energetic tokamaks about the globe, they’re high-priced devices and in substantial demand. For instance, TCV can only maintain the plasma in a solitary experiment for up to 3 seconds, right after which it wants 15 minutes to interesting down and reset prior to the future endeavor. Not only that, several analysis groups frequently share use of the tokamak, further limiting the time accessible for experiments.
Presented the current hurdles to access a tokamak, scientists have turned to simulators to enable progress analysis. For instance, our partners at EPFL have designed a highly effective set of simulation resources that design the dynamics of tokamaks. We were being in a position to use these to allow for our RL technique to understand to management TCV in simulation and then validate our benefits on the genuine TCV, displaying we could productively sculpt the plasma into the wanted styles. Whilst this is a much less expensive and much more handy way to prepare our controllers we nevertheless experienced to prevail over a lot of obstacles. For case in point, plasma simulators are slow and require quite a few several hours of computer system time to simulate a person 2nd of real time. In addition, the ailment of TCV can modify from working day to working day, necessitating us to create algorithmic advancements, each actual physical and simulated, and to adapt to the realities of the hardware.
Success by prioritising simplicity and versatility
Present plasma-control techniques are advanced, requiring separate controllers for every of TCV’s 19 magnetic coils. Just about every controller utilizes algorithms to estimate the properties of the plasma in serious time and adjust the voltage of the magnets appropriately. In distinction, our architecture employs a one neural network to manage all of the coils at when, quickly understanding which voltages are the very best to attain a plasma configuration straight from sensors.
As a demonstration, we 1st confirmed that we could manipulate several elements of the plasma with a solitary controller.

In the video above, we see the plasma at the best of TCV at the prompt our method will take control. Our controller initially styles the plasma in accordance to the requested shape, then shifts the plasma downward and detaches it from the walls, suspending it in the center of the vessel on two legs. The plasma is held stationary, as would be essential to evaluate plasma houses. Then, lastly the plasma is steered back again to the top of the vessel and safely and securely wrecked.
We then created a range of plasma styles currently being examined by plasma physicists for their usefulness in producing power. For case in point, we created a “snowflake” shape with quite a few “legs” that could support cut down the expense of cooling by spreading the exhaust strength to distinct contact points on the vessel partitions. We also shown a condition near to the proposal for ITER, the next-technology tokamak under building, as EPFL was conducting experiments to forecast the behaviour of plasmas in ITER. We even did a little something that experienced by no means been completed in TCV prior to by stabilising a “droplet” where by there are two plasmas inside of the vessel at the same time. Our single technique was equipped to discover controllers for all of these distinct circumstances. We basically altered the purpose we asked for, and our algorithm autonomously discovered an ideal controller.
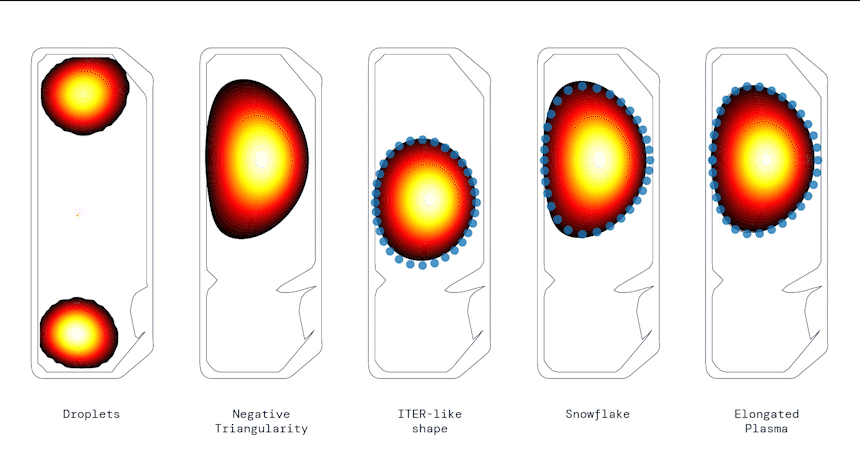
The long run of fusion and outside of
Related to progress we’ve viewed when applying AI to other scientific domains, our productive demonstration of tokamak management displays the electricity of AI to speed up and help fusion science, and we count on escalating sophistication in the use of AI heading forward. This ability of autonomously developing controllers could be utilized to style and design new types of tokamaks while at the same time creating their controllers. Our function also factors to a bright potential for reinforcement finding out in the command of sophisticated machines. It is particularly fascinating to think about fields exactly where AI could increase human know-how, serving as a software to learn new and resourceful ways for tough genuine-entire world problems. We predict reinforcement studying will be a transformative technological innovation for industrial and scientific command apps in the yrs to arrive, with apps ranging from electrical power efficiency to personalised medicine.
[ad_2]
Resource url