[ad_1]
Lots of new successes in language designs (LMs) have been realized inside a ‘static paradigm’, wherever the concentration is on improving efficiency on the benchmarks that are designed without contemplating the temporal factor of data. For occasion, answering issues on functions that the design could understand about for the duration of education, or analyzing on text sub-sampled from the similar period as the education data. Having said that, our language and know-how are dynamic and at any time evolving. Consequently, to allow a additional reasonable analysis of problem-answering products for the future leap in performance, it’s crucial to assure they are versatile and sturdy when encountering new and unseen facts.
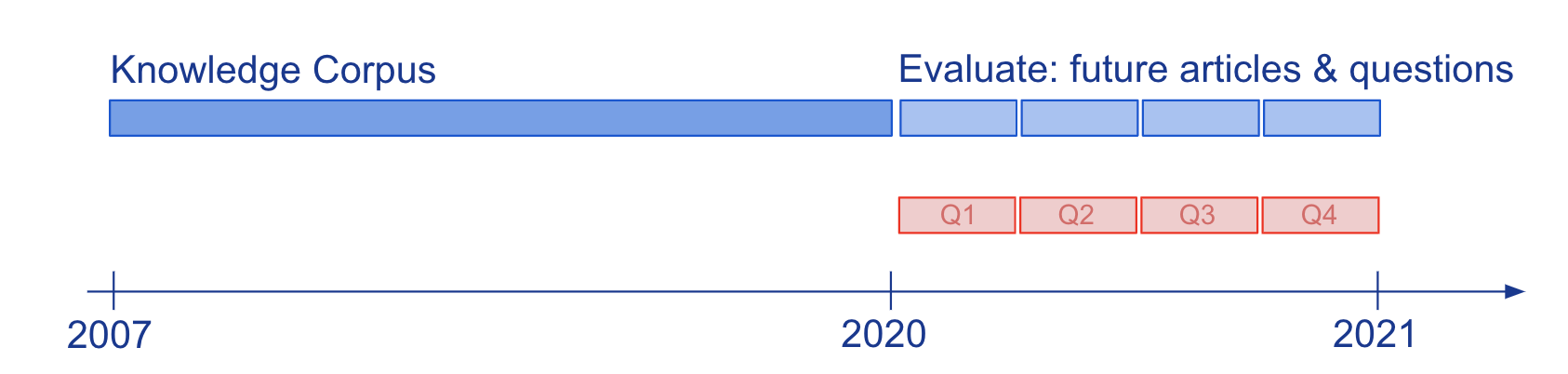
In 2021, we unveiled Thoughts the Hole: Examining Temporal Generalization in Neural Language Models and the dynamic language modelling benchmarks for WMT and arXiv to aid language product evaluation that acquire temporal dynamics into account. In this paper, we highlighted difficulties that latest point out-of-the-artwork substantial LMs experience with temporal generalisation and uncovered that expertise-intense tokens choose a appreciable efficiency hit.
Nowadays, we’re releasing two papers and a new benchmark that more advance study on this subject matter. In StreamingQA: A Benchmark for Adaptation to New Know-how over Time in Concern Answering Types, we review the downstream endeavor of problem-answering on our newly proposed benchmark, StreamingQA: we want to fully grasp how parametric and retrieval-augmented, semi-parametric issue-answering designs adapt to new information and facts, in buy to respond to thoughts about new occasions. In World wide web-augmented language types by way of few-shot prompting for open-domain issue answering, we explore the ability of combining a handful of-shot prompted significant language product along with Google Lookup as a retrieval element. In accomplishing so, we aim to increase the model’s factuality, when earning positive it has accessibility to up-to-day info for answering a varied established of questions.
StreamingQA: A Benchmark for Adaptation to New Expertise over Time in Question Answering Models
Expertise and language comprehension of versions evaluated via dilemma-answering (QA) has been generally researched on static snapshots of know-how, like Wikipedia. To review how semi-parametric QA designs and their fundamental parametric LMs adapt to evolving understanding, we constructed the new large-scale benchmark, StreamingQA, with human-composed and quickly generated inquiries requested on a presented date, to be answered from 14 decades of time-stamped information articles (see Figure 2). We present that parametric versions can be up to date with out full retraining, when steering clear of catastrophic forgetting. For semi-parametric products, incorporating new article content into the research area permits for speedy adaptation, nevertheless, versions with an outdated fundamental LM underperform people with a retrained LM.
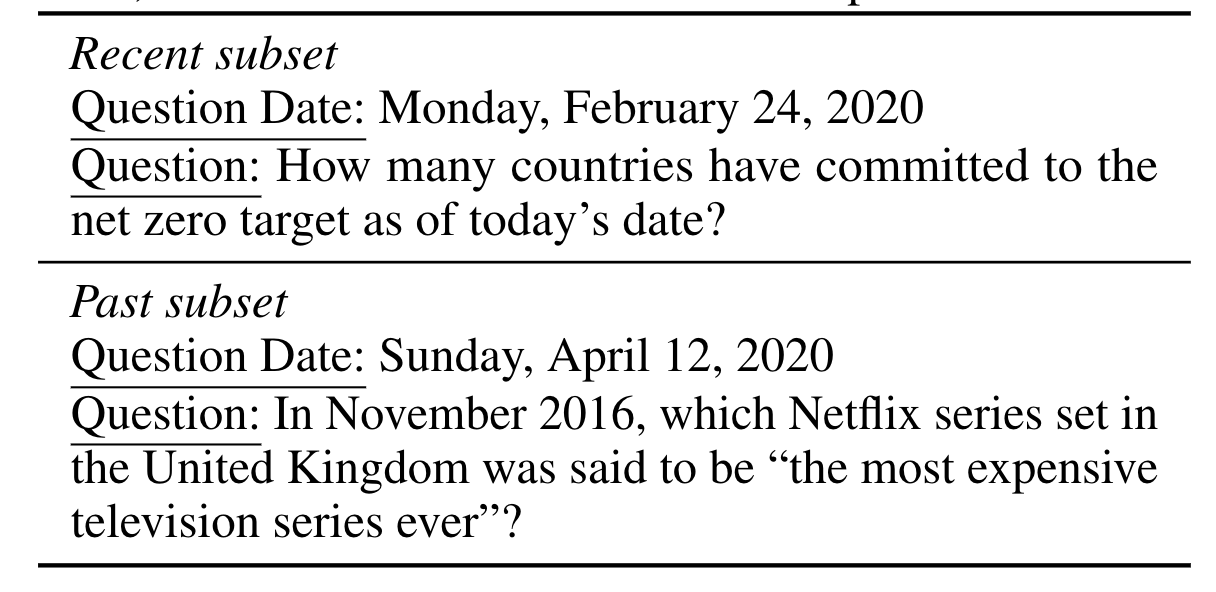
Net-augmented language designs as a result of handful of-shot prompting for open-domain query-answering
We’re aiming to capitalise on the exclusive several-shot capabilities offered by significant-scale language products to get over some of their difficulties, with regard to grounding to factual and up-to-day information and facts. Motivated by semi-parametric LMs, which floor their choices in externally retrieved proof, we use couple of-shot prompting to study to problem LMs on information returned from the internet working with Google Look for, a broad and frequently up to date understanding resource. Our method does not involve high-quality-tuning or discovering additional parameters, therefore creating it applicable to nearly any language model. And without a doubt, we locate that LMs conditioned on the net surpass the performance of shut-reserve versions of comparable, or even greater, design size in open-area question-answering.
[ad_2]
Source backlink