[ad_1]
Locating alternatives to enhance turtle reidentification and supporting device learning assignments throughout Africa
Preserving the ecosystems all-around us is significant to safeguarding the future of our planet and all its dwelling citizens. The good thing is, new artificial intelligence (AI) techniques are producing development in conservation initiatives all over the world, helping tackle intricate challenges at scale – from researching the conduct of animal communities in the Serengeti to assist preserve the diminishing ecosystem, to recognizing poachers and their wounded prey to avert species heading extinct.
As aspect of our mission to help advantage humanity with the systems we create, it can be vital we guarantee varied groups of folks develop the AI techniques of the upcoming so that it is equitable and good. This incorporates broadening the equipment learning (ML) group and partaking with wider audiences on addressing essential issues using AI.
Via investigation, we came throughout Zindi – a dedicated lover with complementary objectives – who are the premier community of African facts scientists and host competitions that focus on solving Africa’s most pressing challenges.
Our Science group’s Variety, Fairness, and Inclusion (DE&I) group labored with Zindi to discover a scientific obstacle that could assist progress conservation initiatives and increase involvement in AI. Motivated by Zindi’s bounding box turtle challenge, we landed on a undertaking with the prospective for actual effects: turtle facial recognition.
Biologists take into consideration turtles to be an indicator species. These are classes of organisms whose conduct assists scientists comprehend the underlying welfare of their ecosystem. For example, the presence of otters in rivers has been thought of a indicator of a clear, wholesome river, because a ban on chlorine pesticides in the 1970s brought the species back from the brink of extinction.
Turtles are a different these types of species. By grazing on seagrass go over, they cultivate the ecosystem, supplying a habitat for numerous fish and crustaceans. Customarily, personal turtles have been discovered and tracked by biologists with actual physical tags, though recurrent reduction or erosion of these tags in seawater has designed this an unreliable strategy. To aid solve some of these worries, we introduced an ML problem known as Turtle Recall.
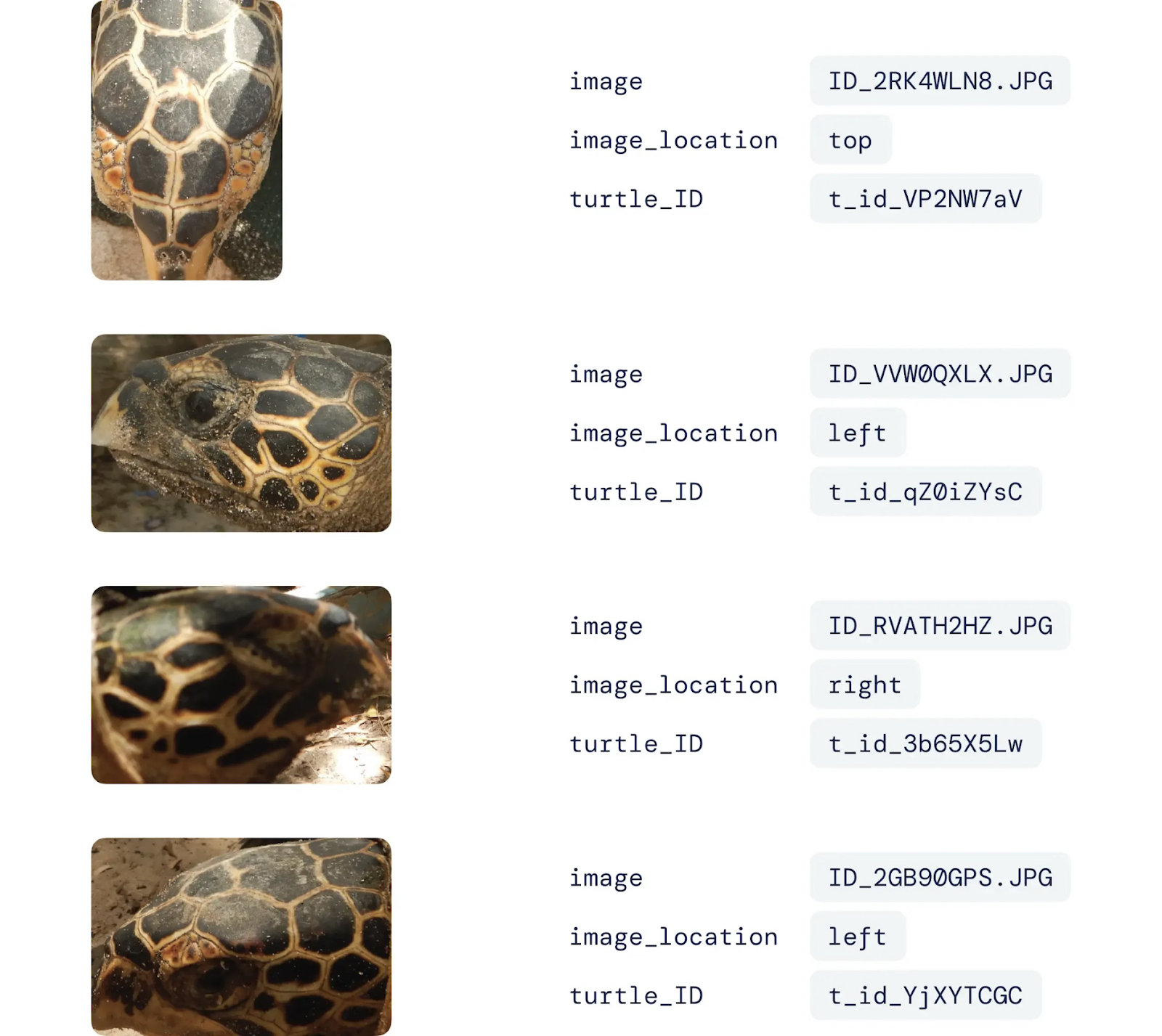
Presented the additional obstacle of trying to keep a turtle continue to plenty of to identify their tag, the Turtle Remember problem aimed to circumvent these issues with turtle facial recognition. This is possible simply because the pattern of scales on a turtle’s face is exclusive to the specific and remains the identical above their multi-decade lifespan.
The problem aimed to raise the dependability and speed of turtle reidentification, and perhaps supply a way to exchange the use of uncomfortable bodily tags altogether. To make this doable, we required a dataset to get the job done from. Thankfully, following Zindi’s prior turtle-based obstacle with Kenyan-based charity Local Ocean Conservation, the teams had been kindly capable to share a dataset of labelled photos of turtle faces.
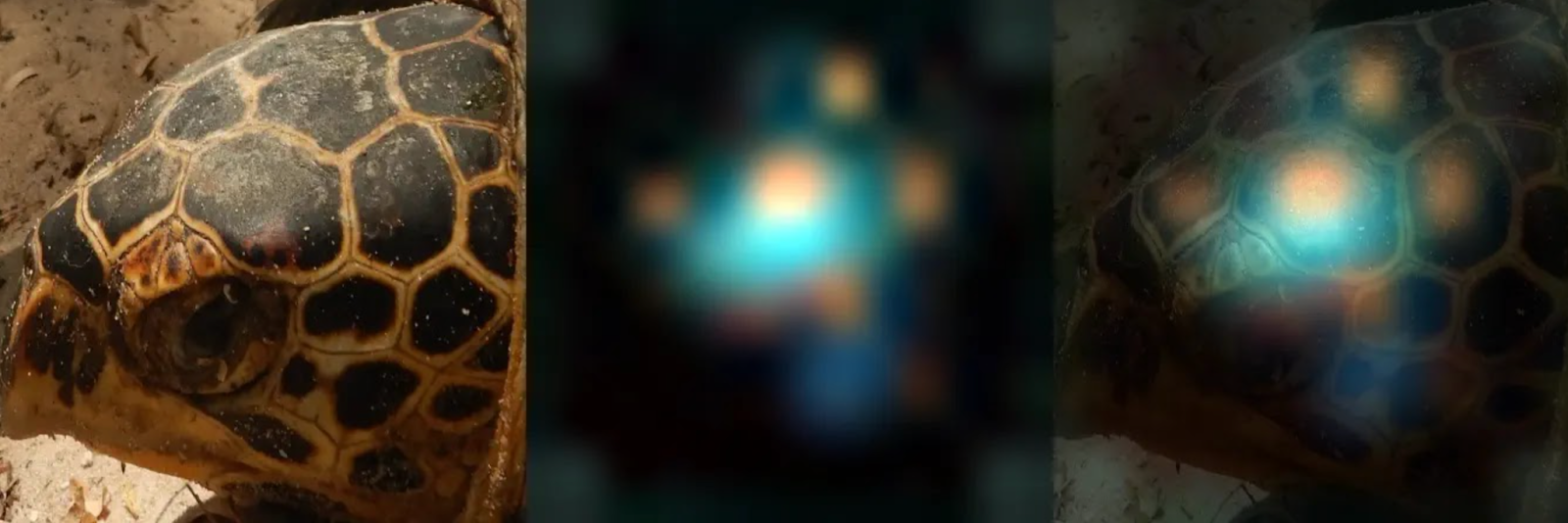
The levels of competition started off in November 2021 and lasted five months. To encourage competitor participation, the team applied a colab notebook, an in-browser programming surroundings, which released two common programming resources: JAX and Haiku.
Members had been tasked with downloading the obstacle data and training products to forecast a turtle’s identification, as accurately as possible, provided a photograph taken from a precise angle. Owning submitted their predictions on information withheld from the product, they had been able to visit a general public leaderboard monitoring the development of every participant.
The group engagement was exceptionally positive, and so was the technical innovation shown by groups throughout the problem. Through the system of the levels of competition, we gained submissions from a various range of AI fans from 13 diverse African international locations – like countries not customarily very well represented at the greatest ML conferences, this kind of as Ghana and Benin.
Our turtle conservation associates have indicated that the participant’s amount of prediction precision will be right away practical for determining turtles in the subject, which means that these products can have a serious and immediate influence on wildlife conservation.
As aspect of Zindi’s continued endeavours to help local weather-optimistic issues, they are also performing on Swahili audio classification in Kenya to assistance translation and unexpected emergency solutions, and air quality prediction in Uganda to enhance social welfare.
We are grateful to Zindi for their partnership, and all all those who contributed their time to the Turtle Recall challenge and the growing field of AI for conservation. And we look forward to looking at how individuals around the planet proceed to obtain methods to utilize AI technologies in direction of building a nutritious, sustainable potential for the earth.
Go through much more about Turtle Recall on Zindi’s web site and study about Zindi at https://zindi.africa/
[ad_2]
Supply connection