[ad_1]
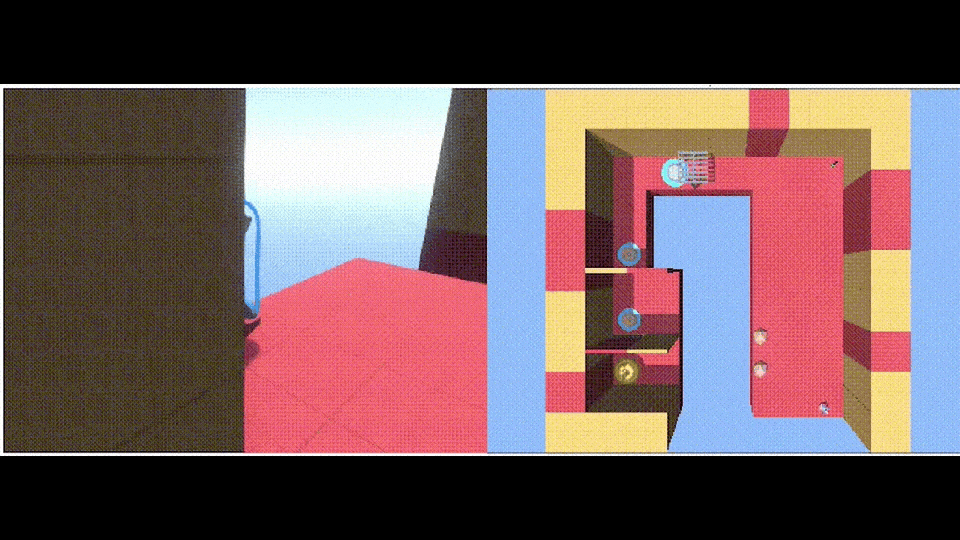
Curiosity-driven exploration is the lively course of action of trying to get new information and facts to improve the agent’s knowing of its environment. Suppose that the agent has figured out a product of the world that can forecast long term situations given the record of earlier gatherings. The curiosity-driven agent can then use the prediction mismatch of the earth product as the intrinsic reward for directing its exploration plan toward searching for new info. As follows, the agent can then use this new details to greatly enhance the world model alone so it can make superior predictions. This iterative procedure can make it possible for the agent to at some point check out each individual novelty in the globe and use this info to establish an correct world product.
Encouraged by the successes of bootstrap your have latent (BYOL) – which has been used in personal computer vision, graph representation studying, and representation discovering in RL – we propose BYOL-Investigate: a conceptually uncomplicated nonetheless standard, curiosity-driven AI agent for solving hard-exploration responsibilities. BYOL-Examine learns a illustration of the planet by predicting its possess foreseeable future illustration. Then, it takes advantage of the prediction-error at the illustration stage as an intrinsic reward to educate a curiosity-pushed policy. For that reason, BYOL-Examine learns a earth representation, the earth dynamics, and a curiosity-driven exploration policy all-together, merely by optimising the prediction mistake at the illustration stage.

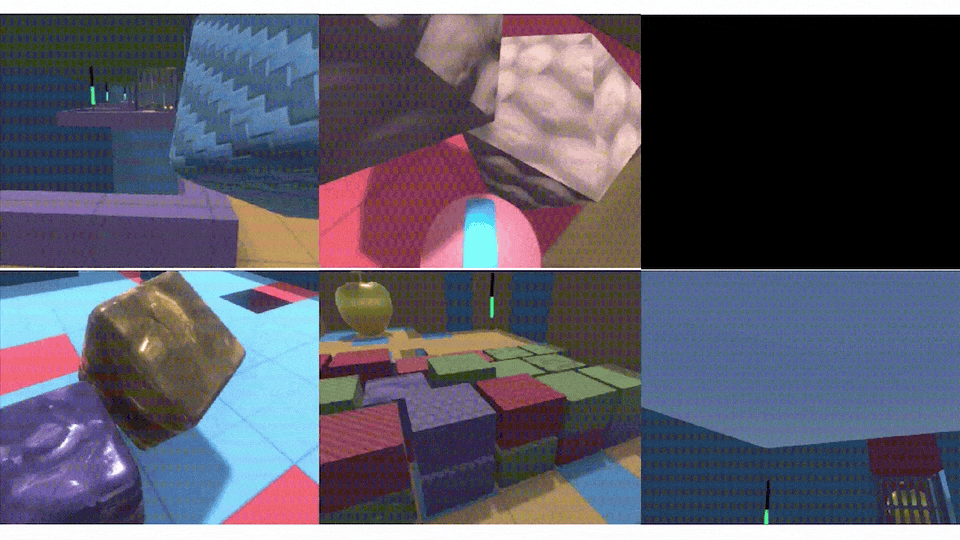
Inspite of the simplicity of its design and style, when utilized to the DM-Really hard-8 suite of tough 3-D, visually intricate, and tricky exploration duties, BYOL-Check out outperforms regular curiosity-driven exploration approaches such as Random Community Distillation (RND) and Intrinsic Curiosity Module (ICM), in phrases of necessarily mean capped human-normalised score (CHNS), calculated throughout all tasks. Remarkably, BYOL-Discover obtained this effectiveness utilizing only a one community concurrently skilled across all responsibilities, whereas prior get the job done was limited to the single-job environment and could only make meaningful progress on these jobs when supplied with human pro demonstrations.
As even more evidence of its generality, BYOL-Explore achieves tremendous-human effectiveness in the ten toughest exploration Atari video games, even though acquiring a less difficult style than other competitive agents, such as Agent57 and Go-Take a look at.

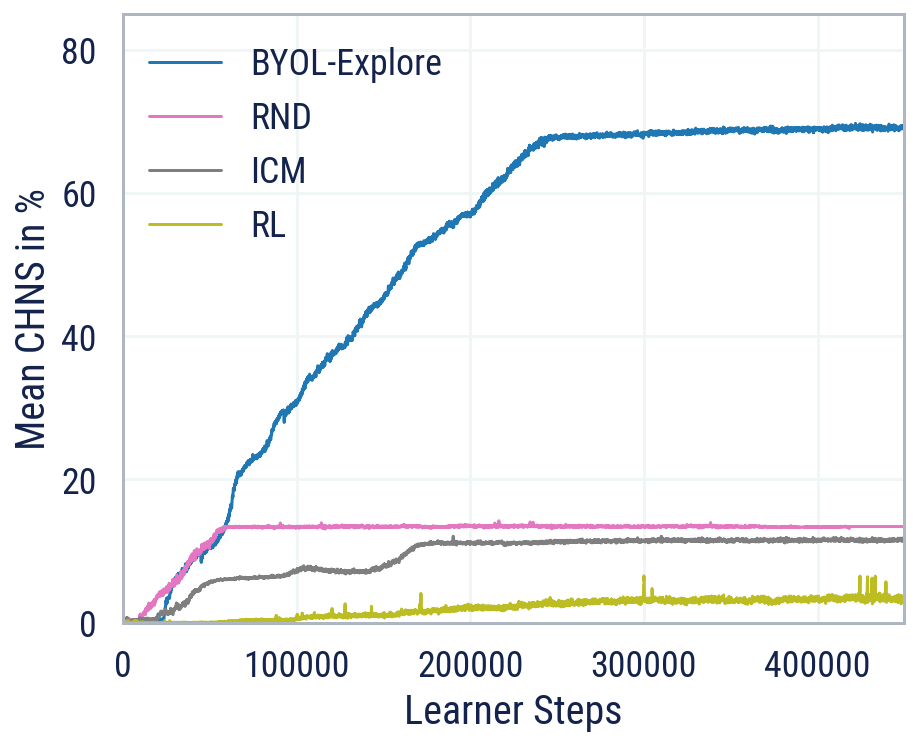
Transferring ahead, we can generalise BYOL-Check out to very stochastic environments by understanding a probabilistic world product that could be used to create trajectories of the long run functions. This could allow the agent to model the attainable stochasticity of the atmosphere, keep away from stochastic traps, and approach for exploration.
[ad_2]
Resource website link