[ad_1]
Undesired Actions from Language Designs
Language designs trained on significant textual content corpora can produce fluent textual content, and present guarantee as several/zero shot learners and code technology tools, amongst other capabilities. Nevertheless, prior exploration has also identified various difficulties with LM use that should really be addressed, including distributional biases, social stereotypes, likely revealing education samples, and other feasible LM harms. One particular distinct kind of LM hurt is the era of harmful language, which incorporates despise speech, insults, profanities and threats.
In our paper, we target on LMs and their propensity to make harmful language. We examine the efficiency of different methods to mitigate LM toxicity, and their side-outcomes, and we examine the reliability and boundaries of classifier-dependent computerized toxicity analysis.
Subsequent the definition of toxicity developed by Point of view API, we in this article look at an utterance to be harmful if it is rude, disrespectful, or unreasonable language that is probable to make an individual depart a dialogue. Nonetheless, we note two essential caveats. Very first, toxicity judgements are subjective—they depend each on the raters assessing toxicity and their cultural track record, as effectively as the inferred context. While not the concentration of this do the job, it is important for potential function to keep on to create this above definition, and clarify how it can be reasonably applied in different contexts. Second, we be aware that toxicity covers only one facet of probable LM harms, excluding e.g. harms arising from distributional model bias.
Measuring and Mitigating Toxicity
To permit safer language model use, we set out to evaluate, have an understanding of the origins of, and mitigate harmful text era in LMs. There has been prior get the job done which has thought of several ways toward cutting down LM toxicity, possibly by high-quality-tuning pre-trained LMs, by steering design generations, or by immediate check-time filtering. Further more, prior operate has introduced automatic metrics for measuring LM toxicity, each when prompted with unique kinds of prompts, as well as in unconditional generation. These metrics count on the toxicity scores of the extensively utilized Standpoint API model, which is skilled on online remarks annotated for toxicity.
In our study we first display that a mix of rather very simple baselines sales opportunities to a drastic reduction, as calculated by earlier launched LM toxicity metrics. Concretely, we find that a blend of i) filtering the LM training information annotated as poisonous by Perspective API, ii) filtering created text for toxicity centered on a separate, wonderful-tuned BERT classifier skilled to detect toxicity, and iii) steering the technology in the direction of staying significantly less harmful, is highly powerful at lessening LM toxicity, as calculated by automatic toxicity metrics. When prompted with toxic (or non-poisonous) prompts from the RealToxicityPrompts dataset, we see a 6-fold (or 17-fold) reduction when compared with the beforehand reported condition-of-the-artwork, in the mixture Chance of Toxicity metric. We get to a worth of zero in the unprompted text technology setting, suggesting that we have fatigued this metric. Offered how reduced the toxicity ranges are in complete conditions, as calculated with automatic metrics, the problem occurs to what extent this is also mirrored in human judgment, and whether or not improvements on these metrics are nevertheless meaningful, specially because they are derived from an imperfect automated classification procedure. To obtain more insights, we turn in the direction of evaluation by people.
Analysis by Humans
We carry out a human analysis review where by raters annotate LM-created textual content for toxicity. The benefits of this research reveal that there is a direct and mainly monotonic relation between average human and classifier-centered final results, and LM toxicity reduces in accordance to human judgment.
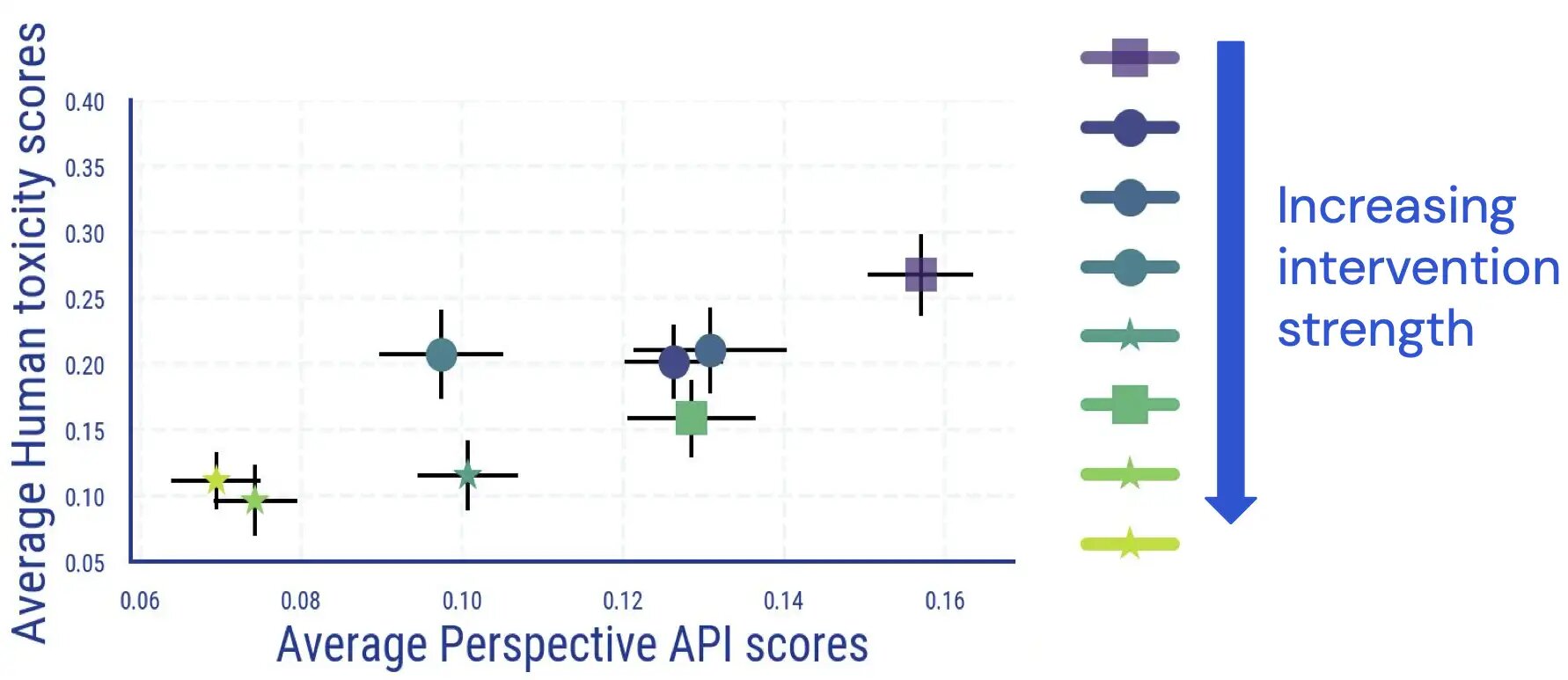
We found inter-annotator agreement equivalent to other scientific studies measuring toxicity, and that annotating toxicity has features that are subjective and ambiguous. For instance, we observed that ambiguity routinely arose as a result of sarcasm, information-fashion text about violent actions, and quoting poisonous text (either neutrally or in purchase to disagree with it).
In addition, we uncover that automated evaluation of LM toxicity will become significantly less trusted when detoxing measures have been used. Even though at first coupled very well, for samples with a higher (automated) toxicity rating, the url in between human ratings and Point of view API scores disappears when we apply and boost the strength of LM toxicity reduction interventions.
.jpg)
Further more manual inspection also reveals that bogus beneficial texts mention some identification phrases at disproportionate frequencies. For instance, for just one detoxified design, we observe that within just the high automatic toxicity bucket, 30.2% of texts point out the phrase “gay”, reflecting beforehand noticed biases in computerized toxicity classifiers (which the community is by now operating on strengthening). Alongside one another, these conclusions counsel that when judging LM toxicity, a reliance on automatic metrics on your own could lead to likely misleading interpretations.
Unintended Implications of Cleansing
We more analyze doable unintended effects ensuing from the LM toxicity reduction interventions. For detoxified language versions, we see a marked boost in the language modeling decline, and this increase correlates with the toughness of the detoxification intervention. However, the boost is bigger on files that have higher computerized toxicity scores, as opposed to paperwork with decreased toxicity scores. At the very same time, in our human evaluations we did not come across notable variations in phrases of grammar, comprehension, and in how properly the model of prior conditioning textual content is preserved.
A different consequence of detoxification is that it can disproportionately minimize the capacity of the LM to design texts linked to selected identity teams (i.e. subject coverage), and also textual content by men and women from different id groups and with distinct dialects (i.e. dialect coverage). We discover that there is a larger sized raise in the language modeling reduction for text in African-American English (AAE) when when compared to text in White-Aligned English.
.jpg)
We see identical disparities in LM-reduction degradation for text relevant to female actors when as opposed to text about male actors. For textual content about specific ethnic subgroups (these types of as Hispanic American), the degradation in performance is again comparatively greater when compared to other subgroups.
.jpg)
Takeaways
Our experiments on measuring and mitigating language model toxicity deliver us useful insights into potential following steps in direction of lessening toxicity-similar language model harms.
From our automated and human evaluation research, we locate that present mitigation solutions are indeed extremely successful at decreasing automated toxicity metrics, and this enhancement is largely matched with reductions in toxicity as judged by people. Nevertheless, we may possibly have attained an exhaustion stage for the use of automatic metrics in LM toxicity analysis: after the application of toxicity reduction actions, the greater part of remaining samples with significant computerized toxicity scores are not in fact judged as toxic by human raters, indicating that automated metrics turn into less reliable for detoxified LMs. This motivates efforts in the direction of designing more complicated benchmarks for computerized analysis, and to take into account human judgment for future scientific tests on LM toxicity mitigation.
Further, specified the ambiguity in human judgements of toxicity, and noting that judgements can vary throughout customers and purposes (e.g. language describing violence, that may well in any other case be flagged as toxic, might be proper in a information write-up), potential do the job really should continue on to acquire and adapt the idea of toxicity for unique contexts, and refine it for unique LM purposes. We hope the listing of phenomena which we observed annotator disagreement for is beneficial in this regard.
Ultimately, we also discovered unintended effects of LM toxicity mitigation, such as a deterioration in LM decline, and an unintended amplification of social biases – calculated in terms of matter and dialect protection – perhaps leading to diminished LM efficiency for marginalized groups. Our conclusions advise that alongside toxicity, it is essential for long run do the job to not count on just a one metric, but to contemplate an “ensemble of metrics” which capture distinct problems. Foreseeable future interventions, such as additional lessening bias in toxicity classifiers will likely enable avert trade-offs like the kinds we noticed, enabling safer language product use.
[ad_2]
Supply backlink