[ad_1]
In our new paper, we discover how populations of deep reinforcement learning (deep RL) agents can learn microeconomic behaviours, these types of as creation, use, and buying and selling of merchandise. We obtain that artificial agents learn to make economically rational choices about production, intake, and price ranges, and respond correctly to source and need modifications. The population converges to neighborhood charges that reflect the nearby abundance of assets, and some brokers find out to transport merchandise concerning these places to “buy reduced and offer high”. This do the job developments the broader multi-agent reinforcement mastering analysis agenda by introducing new social issues for agents to study how to address.
Insofar as the objective of multi-agent reinforcement understanding analysis is to finally develop brokers that get the job done throughout the whole vary and complexity of human social intelligence, the set of domains so significantly viewed as has been woefully incomplete. It is continue to lacking vital domains wherever human intelligence excels, and individuals commit significant amounts of time and electricity. The subject matter matter of economics is one such domain. Our purpose in this function is to set up environments centered on the themes of investing and negotiation for use by scientists in multi-agent reinforcement mastering.
Economics makes use of agent-centered versions to simulate how economies behave. These agent-dependent types generally create in financial assumptions about how brokers must act. In this perform, we existing a multi-agent simulated entire world where by agents can study economic behaviours from scratch, in approaches common to any Microeconomics 101 pupil: choices about production, use, and selling prices. But our brokers also will have to make other alternatives that comply with from a much more physically embodied way of contemplating. They must navigate a bodily surroundings, locate trees to decide on fruits, and associates to trade them with. Recent advancements in deep RL methods now make it possible to develop agents that can study these behaviours on their individual, without having necessitating a programmer to encode area expertise.
Our atmosphere, termed Fruit Sector, is a multiplayer atmosphere the place brokers deliver and eat two styles of fruit: apples and bananas. Every agent is qualified at manufacturing one variety of fruit, but has a choice for the other – if the brokers can understand to barter and exchange products, both equally functions would be far better off.
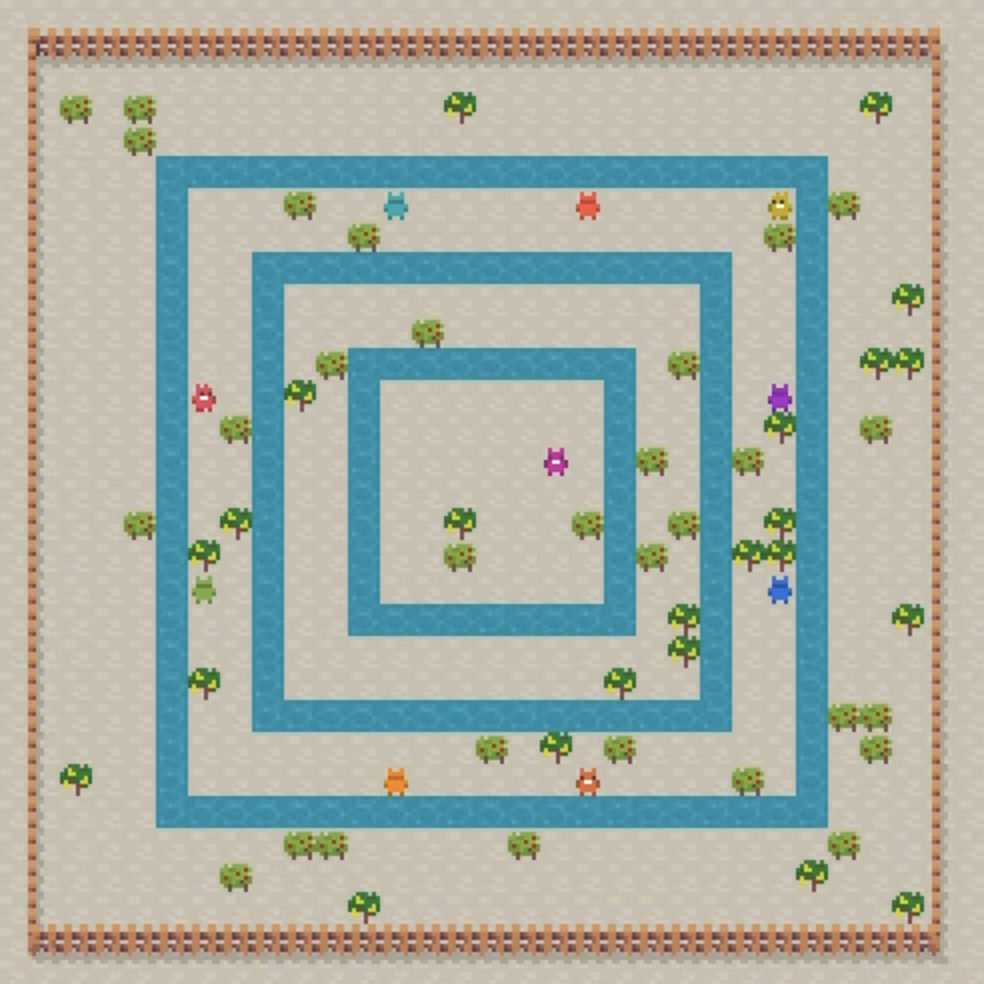
In our experiments, we demonstrate that current deep RL agents can understand to trade, and their behaviours in reaction to offer and need shifts align with what microeconomic idea predicts. We then develop on this function to existing situations that would be incredibly challenging to solve making use of analytical types, but which are clear-cut for our deep RL brokers. For instance, in environments in which each variety of fruit grows in a distinct region, we notice the emergence of distinct cost areas related to the nearby abundance of fruit, as properly as the subsequent mastering of arbitrage conduct by some agents, who commence to specialise in transporting fruit involving these areas.
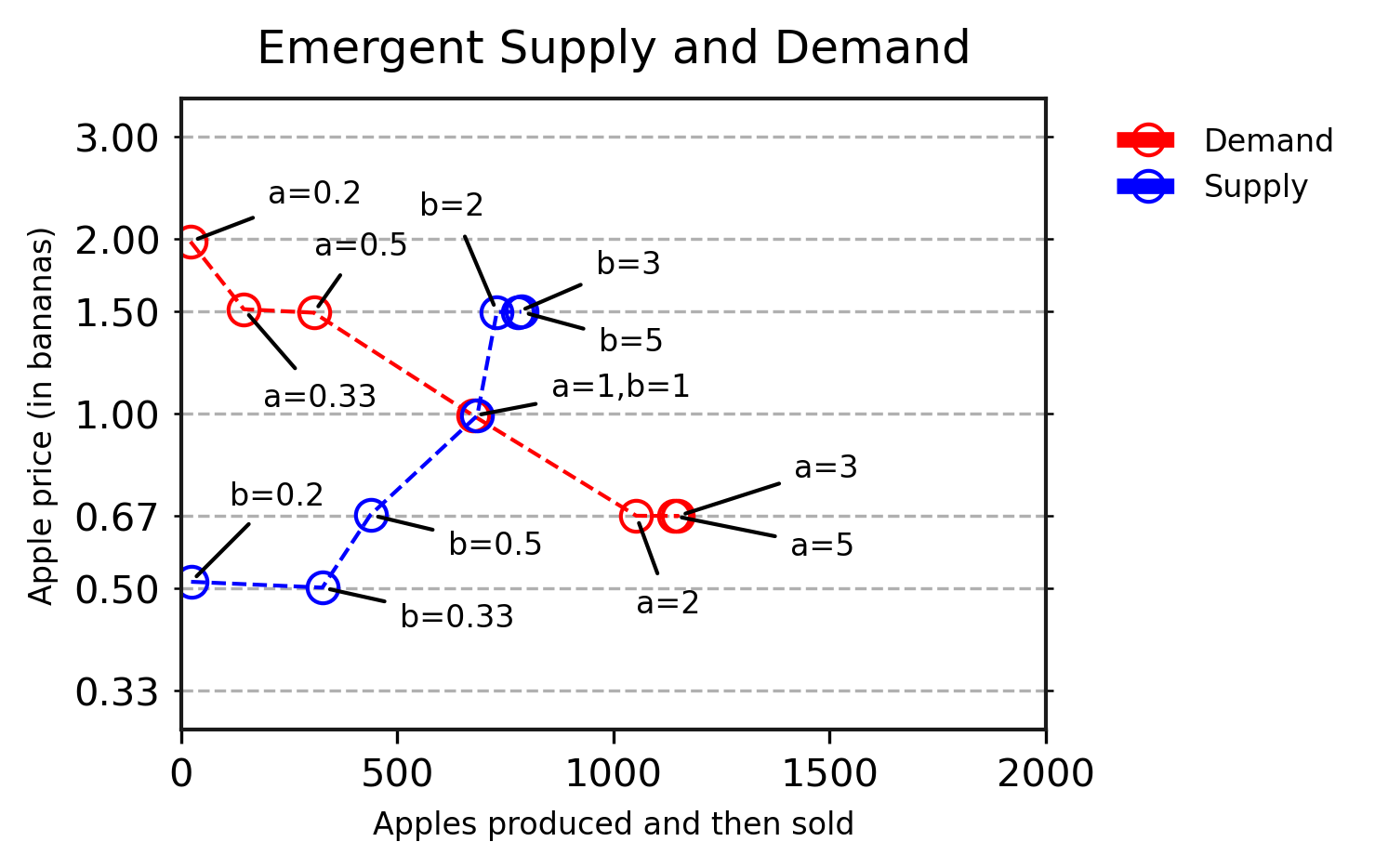
The subject of agent-primarily based computational economics utilizes comparable simulations for economics investigation. In this perform, we also display that point out-of-the-art deep RL procedures can flexibly study to act in these environments from their have experience, without having needing to have economic awareness developed in. This highlights the reinforcement studying community’s current development in multi-agent RL and deep RL, and demonstrates the possible of multi-agent methods as instruments to progress simulated economics research.
As a route to artificial common intelligence (AGI), multi-agent reinforcement mastering research need to encompass all significant domains of social intelligence. Nonetheless, right up until now it has not incorporated regular economic phenomena these kinds of as trade, bargaining, specialisation, intake, and manufacturing. This paper fills this hole and gives a platform for additional investigate. To support foreseeable future analysis in this place, the Fruit Current market atmosphere will be incorporated in the next release of the Melting Pot suite of environments.
[ad_2]
Supply link