[ad_1]
Reflections and classes on sharing a single of our greatest breakthroughs with the earth
Placing our mission of resolving intelligence to progress science and advantage humanity into apply comes with crucial responsibilities. To support develop a positive impression for culture, we ought to proactively assess the ethical implications of our investigation and its applications in a arduous and careful way. We also know that each individual new engineering has the likely for damage, and we choose prolonged and short expression threats seriously. We have created our foundations on groundbreaking responsibly from the outset – primarily focused on accountable governance, investigation, and impact.
This begins with placing crystal clear principles that enable realise the positive aspects of synthetic intelligence (AI), even though mitigating its challenges and likely negative outcomes. Groundbreaking responsibly is a collective exertion, which is why we have contributed to numerous AI community requirements, these as those formulated by Google, the Partnership on AI, and the OECD (Organisation for Financial Co-operation and Development).
Our Working Principles have occur to determine both equally our motivation to prioritising widespread gain, as properly as the areas of investigate and programs we refuse to pursue. These concepts have been at the coronary heart of our conclusion earning considering that DeepMind was launched, and go on to be refined as the AI landscape variations and grows. They are built for our purpose as a research-pushed science organization and constant with Google’s AI Rules.
From ideas to follow
Composed concepts are only section of the puzzle – how they’re place into exercise is critical. For intricate exploration staying finished at the frontiers of AI, this provides substantial problems: How can scientists predict likely gains and harms that may possibly occur in the distant long run? How can we acquire far better ethical foresight from a wide assortment of perspectives? And what does it consider to discover hard queries along with scientific development in realtime to avoid detrimental repercussions?
We have put in many decades acquiring our possess techniques and procedures for liable governance, research, and affect throughout DeepMind, from developing internal toolkits and publishing papers on sociotechnical troubles to supporting initiatives to raise deliberation and foresight throughout the AI area. To assistance empower DeepMind groups to pioneer responsibly and safeguard versus damage, our interdisciplinary Institutional Evaluation Committee (IRC) satisfies just about every two weeks to thoroughly assess DeepMind assignments, papers, and collaborations.
Groundbreaking responsibly is a collective muscle mass, and just about every task is an chance to strengthen our joint skills and knowledge. We have carefully built our evaluate process to include rotating specialists from a huge range of disciplines, with machine studying scientists, ethicists, and basic safety authorities sitting down alongside engineers, protection professionals, plan gurus, and a lot more. These varied voices routinely recognize ways to develop the benefits of our systems, advise places of investigation and apps to adjust or sluggish, and highlight jobs the place even further external session is required.
Whilst we have produced a large amount of development, a lot of facets of this lie in uncharted territory. We will not get it suitable each individual time and are committed to continual discovering and iteration. We hope sharing our current method will be useful to some others doing work on liable AI, and motivate comments as we proceed to master, which is why we have in-depth reflections and classes from a single of our most sophisticated and fulfilling projects: AlphaFold. Our AlphaFold AI program solved the 50-yr-old problem of protein composition prediction – and we’ve been thrilled to see scientists making use of it to speed up development in fields these kinds of as sustainability, meals protection, drug discovery, and fundamental human biology considering that releasing it to the broader neighborhood past calendar year.
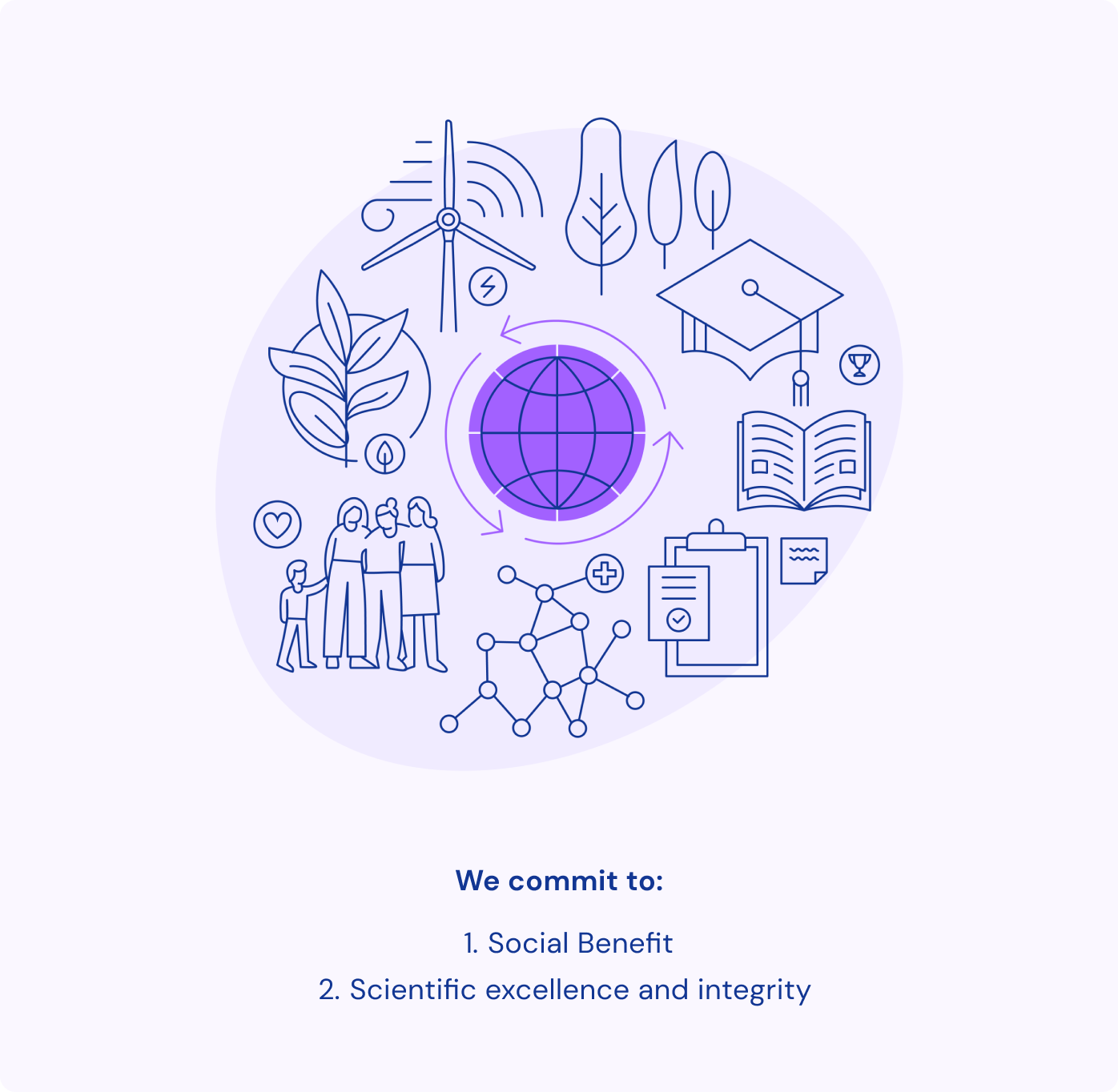
Concentrating on protein framework prediction
Our workforce of device discovering scientists, biologists, and engineers experienced extensive witnessed the protein-folding problem as a amazing and exclusive chance for AI-learning systems to build a sizeable impact. In this arena, there are common steps of good results or failure, and a distinct boundary to what the AI system requirements to do to assist scientists in their do the job – predict the a few-dimensional framework of a protein. And, as with many organic techniques, protein folding is much far too intricate for any one to publish the policies for how it functions. But an AI method may be ready to learn people policies for by itself.
One more critical component was the biennial assessment, acknowledged as CASP (the Essential Assessment of protein Construction Prediction), which was started by Professor John Moult and Professor Krzysztof Fidelis. With just about every gathering, CASP provides an exceptionally strong assessment of progress, demanding members to forecast constructions that have only just lately been found out via experiments. The benefits are a wonderful catalyst for bold investigation and scientific excellence.
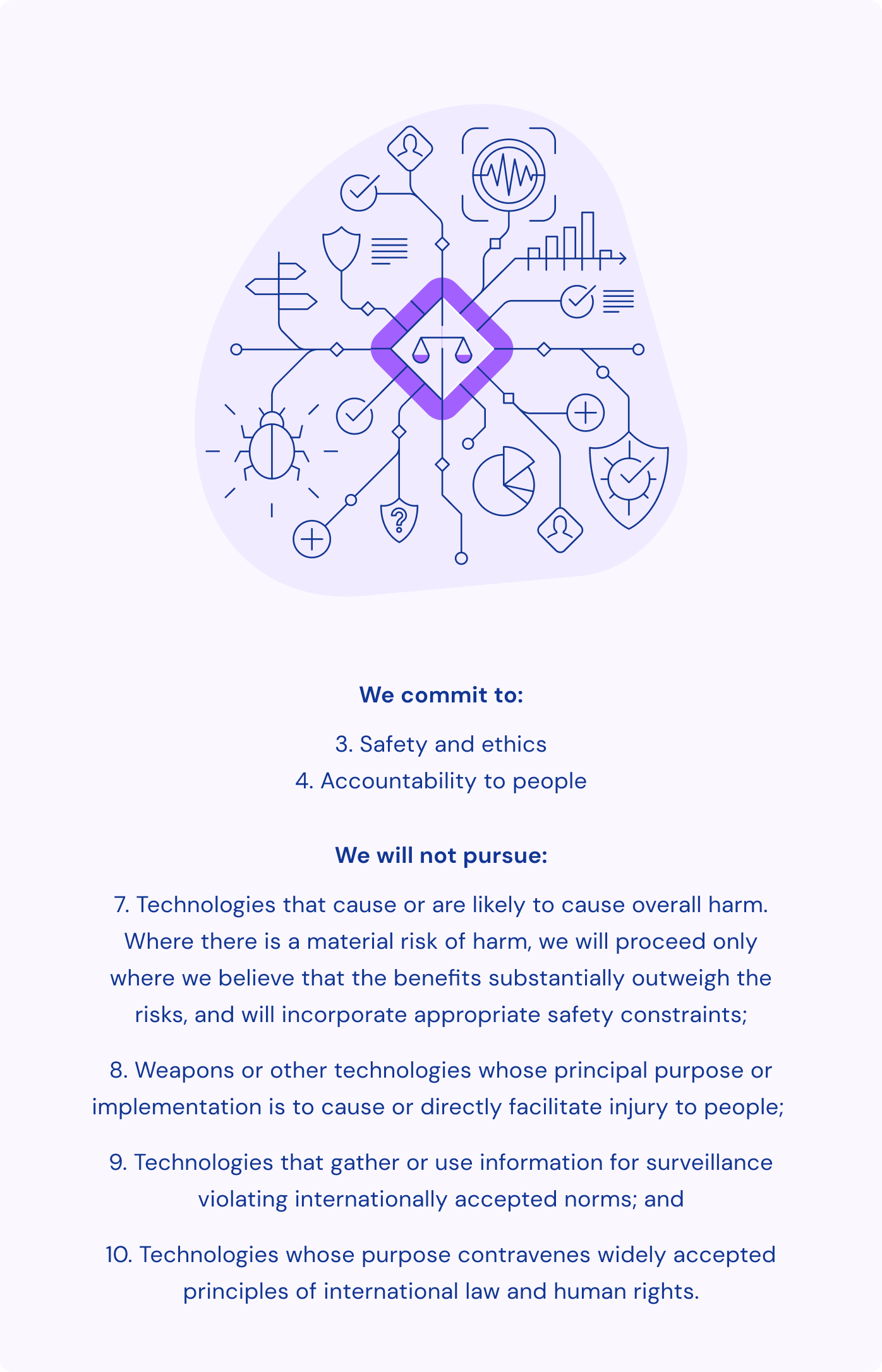
Knowing functional prospects and threats
As we well prepared for the CASP assessment in 2020, we realised that AlphaFold confirmed excellent possible for solving the challenge at hand. We put in substantial time and energy analysing the functional implications, questioning: How could AlphaFold speed up biological investigation and applications? What may well be the unintended effects? And how could we share our development in a dependable way?
This presented a extensive array of possibilities and dangers to contemplate, a lot of of which ended up in places in which we did not essentially have powerful experience. So we sought out external enter from above 30 field leaders throughout biology investigation, biosecurity, bioethics, human rights, and additional, with a concentration on variety of knowledge and history.
Lots of constant themes came up during these discussions:
- Balancing common advantage with the threat of hurt. We started with a careful attitude about the threat of accidental or deliberate damage, including how AlphaFold might interact with each long run advances and present systems. By way of our conversations with external gurus, it grew to become clearer that AlphaFold would not make it meaningfully less complicated to cause damage with proteins, specified the lots of practical obstacles to this – but that long run advances would will need to be evaluated diligently. Lots of experts argued strongly that AlphaFold, as an advance suitable to lots of regions of scientific investigation, would have the best profit as a result of free and common accessibility.
- Correct self confidence steps are necessary for liable use. Experimental biologists spelled out how crucial it would be to realize and share nicely-calibrated and usable self-assurance metrics for each and every element of AlphaFold’s predictions. By signalling which of AlphaFold’s predictions are probably to be exact, end users can estimate when they can belief a prediction and use it in their perform – and when they ought to use substitute strategies in their research. We experienced at first considered omitting predictions for which AlphaFold had minimal self-assurance or significant predictive uncertainty, but the external industry experts we consulted proved why this was primarily vital to keep these predictions in our release, and suggested us on the most practical and transparent techniques to present this details.
- Equitable benefit could mean excess assist for underfunded fields. We had many conversations about how to stay clear of inadvertently escalating disparities inside the scientific local community. For example, so-referred to as neglected tropical ailments, which disproportionately impact poorer components of the world, normally obtain much less investigate funding than they ought to. We were strongly encouraged to prioritise fingers-on guidance and proactively look to lover with teams functioning on these regions.
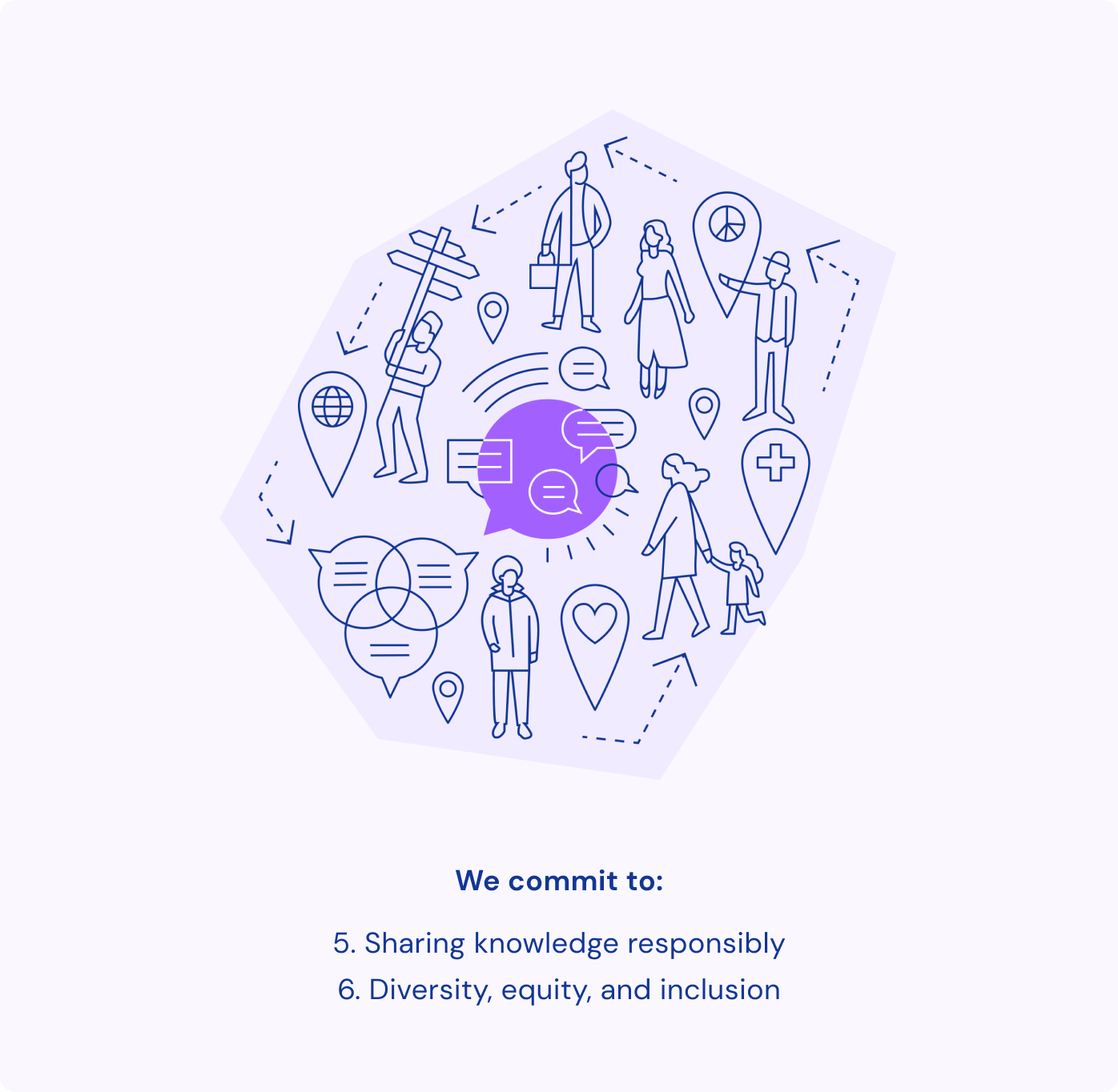
Setting up our release approach
Primarily based on the input over, the IRC endorsed a set of AlphaFold releases to deal with a number of demands, which include:
- Peer-reviewed publications and open source code, together with two papers in Character, accompanied by open up source code, to permit scientists to a lot more easily apply and improve on AlphaFold. Before long after, we included a Google Colab allowing for any person to input a protein sequence and receive a predicted framework, as an substitute to operating the open up resource code themselves.
- A important launch of protein composition predictions in partnership with EMBL-EBI (EMBL’s European Bioinformatics Institute), the established community chief. As a community institution, EMBL-EBI permits everyone to glance up protein composition predictions as effortlessly as a Google research. The initial release incorporated predicted styles for each and every protein in the human system, and our most current update included predicted buildings for nearly all catalogued proteins known to science. This totals more than 200 million buildings, all freely offered on EMBL-EBI’s web-site with open up obtain licences, accompanied by support means, this sort of as webinars on deciphering these constructions.
- Developing 3D visualisations into the database, with prominent labelling for substantial-assurance and small-self esteem areas of the prediction, and, in normal, aiming to be as clear as attainable about AlphaFold’s strengths and limitations in our documentation. We also designed the database to be as obtainable as attainable, for illustration, considering the desires of folks with color vision deficiency.
- Forming further partnerships with analysis groups doing work on underfunded spots, this kind of as neglected health conditions and subject areas important to world wide health. This includes DNDi (Medications for Neglected Disorder initiative), which is advancing research into Chagas sickness and leishmaniasis, and the Centre for Enzyme Innovation which is creating plastic-ingesting enzymes to support minimize plastic squander in the natural environment. Our expanding public engagement teams are continuing to function on these partnerships to help much more collaborations in the long run.
How we’re constructing upon this perform
Considering that our original release, hundreds of hundreds of people today from in excess of 190 nations around the world have frequented the AlphaFold Protein Composition Databases and employed the AlphaFold open up source code because launch. We’ve been honoured to hear of strategies in which AlphaFold’s predictions have accelerated vital scientific endeavours and are operating to notify some of these stories with our Unfolded venture. So much, we’re not informed of any misuse or damage similar to AlphaFold, although we continue to shell out near attention to this.
Whilst AlphaFold was a lot more complex than most DeepMind research tasks, we’re utilizing components of what we’ve acquired and incorporating this into other releases.
We’re making on this work by:
- Growing the selection of enter from external professionals at each individual phase of the procedure, and exploring mechanisms for participatory ethics at higher scale.
- Widening our knowing of AI for biology in general, over and above any personal undertaking or breakthrough, to build a much better see of the alternatives and threats more than time.
- Locating methods to extend our partnerships with groups in fields that are underserved by present-day structures.
Just like our investigation, this is a approach of continuous finding out. The improvement of AI for common reward is a group exertion that spans considerably further than DeepMind.
We’re generating just about every effort and hard work to be mindful of how a great deal difficult get the job done there even now is to do in partnership with many others – and how we pioneer responsibly likely ahead.
[ad_2]
Supply website link