[ad_1]
Grounding language to eyesight is a fundamental trouble for lots of true-globe AI techniques these as retrieving visuals or making descriptions for the visually impaired. Good results on these responsibilities necessitates versions to relate unique aspects of language these as objects and verbs to illustrations or photos. For example, to distinguish concerning the two pictures in the middle column under, styles need to differentiate involving the verbs “catch” and “kick.” Verb knowing is notably tough as it involves not only recognising objects, but also how diverse objects in an image relate to just about every other. To conquer this problem, we introduce the SVO-Probes dataset and use it to probe language and eyesight designs for verb understanding.
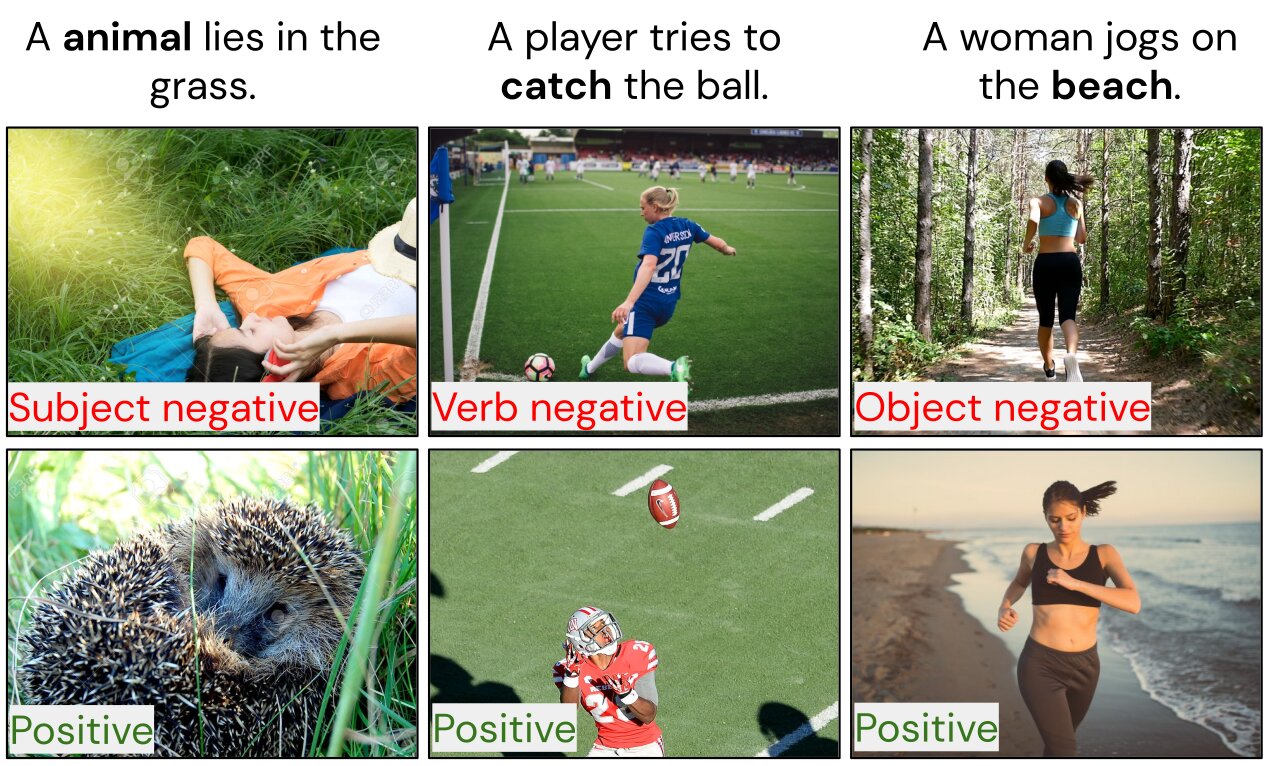
In individual, we consider multimodal transformer products (e.g., Lu et al., 2019 Chen et al., 2020 Tan and Bansal, 2019 Li et al., 2020), which have demonstrated accomplishment on a assortment of language and eyesight jobs. However, regardless of sturdy efficiency on benchmarks, it is not distinct if these types have good-grained multimodal being familiar with. In individual, prior get the job done demonstrates that language and eyesight designs can triumph at benchmarks with no multimodal knowledge: for illustration, answering questions about visuals primarily based only on language priors (Agrawal et al., 2018) or “hallucinating” objects that are not in the image when captioning photos (Rohrbach et al., 2018). To foresee design restrictions, operate like Shekhar et al. suggest specialised evaluations to probe models systematically for language being familiar with. Having said that, prior probe sets are minimal in the variety of objects and verbs. We created SVO-Probes to far better assess prospective limitations in verb comprehending in current types.
SVO-Probes incorporates 48,000 graphic-sentence pairs and tests understanding for more than 400 verbs. Every single sentence can be broken into a
To make SVO-Probes, we question an image search with SVO triplets from a common education dataset, Conceptual Captions (Sharma et al. 2018). Because impression look for can be noisy, a preliminary annotation move filters the retrieved images to ensure we have a thoroughly clean set of picture-SVO pairs. Since transformers are qualified on image-sentence pairs, not impression-SVO pairs, we require impression-sentence pairs to probe our model. To collect sentences which explain every single impression, annotators produce a shorter sentence for each individual impression that consists of the SVO triplet. For instance, specified the SVO triplet
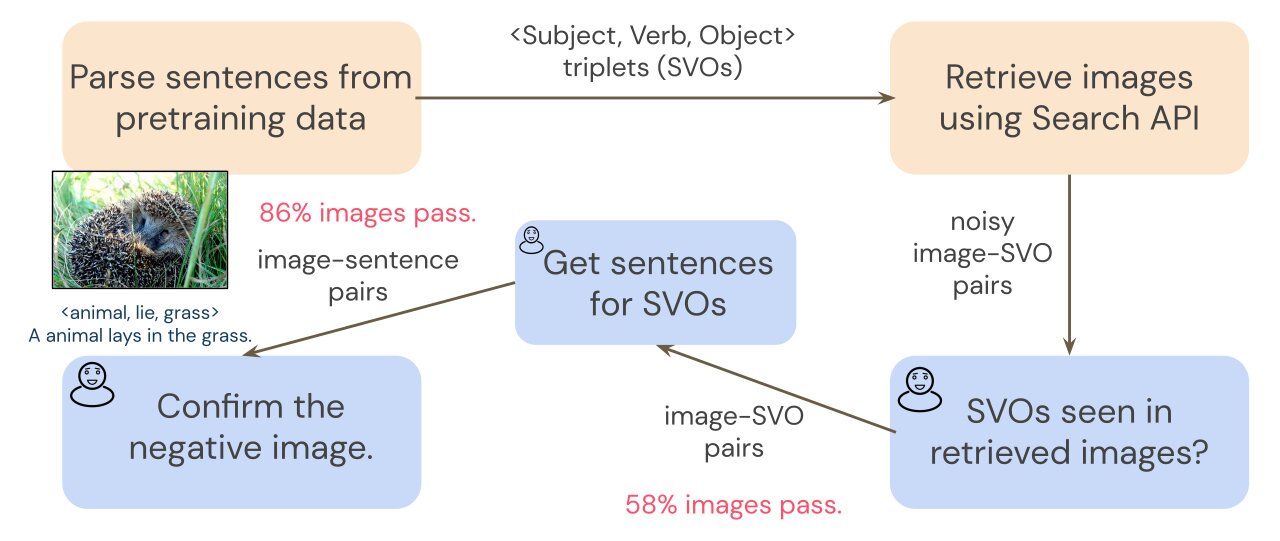
We analyze no matter whether multimodal transformers can correctly classify illustrations as constructive or adverse. The bar chart under illustrates our results. Our dataset is tough: our conventional multimodal transformer product achieves 64.3% accuracy general (chance is 50%). While accuracy is 67.% and 73.4% on topics and objects respectively, general performance falls to 60.8% on verbs. This consequence shows that verb recognition is in fact difficult for eyesight and language products.
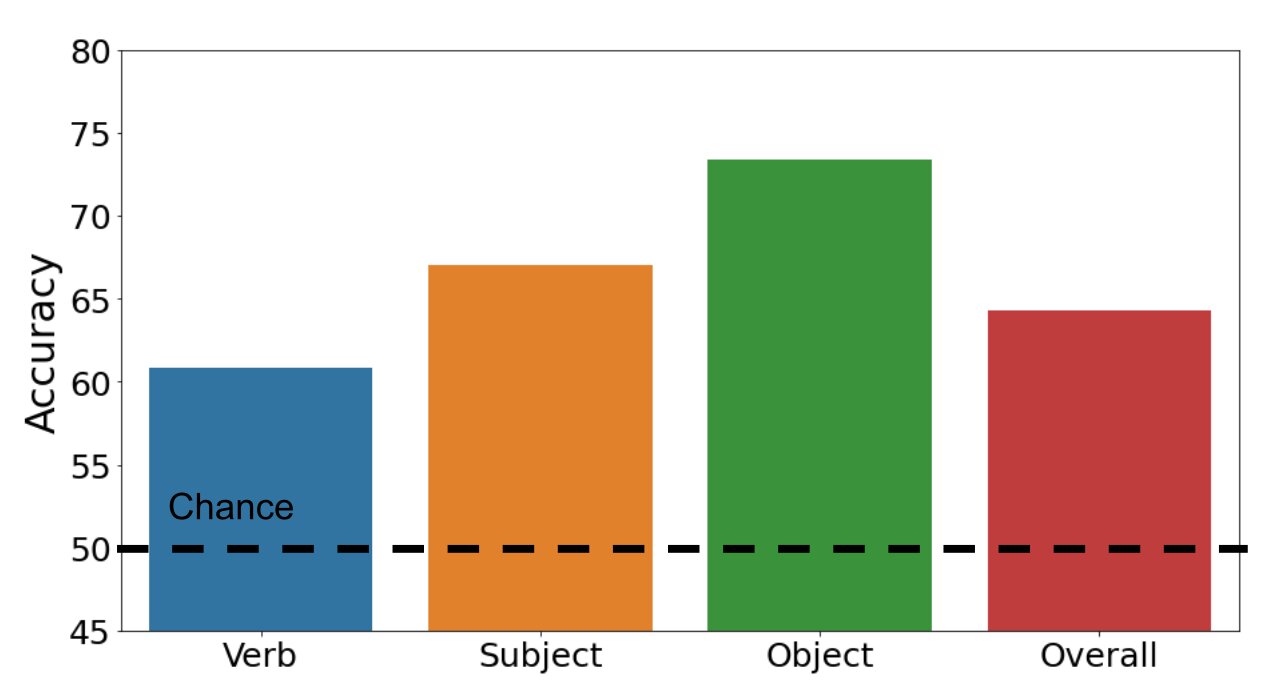
We also take a look at which design architectures execute most effective on our dataset. Astonishingly, designs with weaker impression modeling conduct much better than the standard transformer model. A single speculation is that our common model (with more robust picture modeling capacity) overfits the prepare established. As both these types execute worse on other language and eyesight tasks, our targeted probe undertaking illuminates product weaknesses that are not observed on other benchmarks.
Over-all, we come across that irrespective of spectacular functionality on benchmarks, multimodal transformers even now struggle with great-grained being familiar with, particularly fantastic-grained verb comprehending. We hope SVO-Probes can assist drive exploration of verb knowledge in language and vision styles and inspire far more qualified probe datasets.
Pay a visit to our SVO-Probes benchmark and styles on GitHub: benchmark and designs.
[ad_2]
Resource backlink