[ad_1]
New foundation agent learns to function unique robotic arms, solves tasks from as couple as 100 demonstrations, and increases from self-created information.
Robots are quickly turning into part of our day-to-day life, but they are normally only programmed to accomplish precise responsibilities perfectly. Whilst harnessing modern developments in AI could guide to robots that could aid in many far more means, progress in constructing standard-goal robots is slower in aspect for the reason that of the time wanted to collect serious-planet schooling data.
Our newest paper introduces a self-bettering AI agent for robotics, RoboCat, that learns to complete a assortment of duties across different arms, and then self-generates new schooling facts to make improvements to its procedure.
Earlier investigation has explored how to acquire robots that can study to multi-job at scale and blend the knowing of language products with the serious-planet capabilities of a helper robot. RoboCat is the initially agent to clear up and adapt to various jobs and do so throughout unique, real robots.
RoboCat learns much a lot quicker than other condition-of-the-art styles. It can decide up a new job with as handful of as 100 demonstrations since it draws from a significant and numerous dataset. This capability will assist accelerate robotics exploration, as it reduces the will need for human-supervised teaching, and is an important stage towards creating a common-purpose robot.
How RoboCat enhances alone
RoboCat is based on our multimodal design Gato (Spanish for “cat”), which can procedure language, images, and actions in both of those simulated and physical environments. We blended Gato’s architecture with a big training dataset of sequences of photos and steps of many robot arms fixing hundreds of diverse jobs.
Right after this very first round of teaching, we introduced RoboCat into a “self-improvement” training cycle with a established of previously unseen responsibilities. The studying of every single new endeavor followed five steps:
- Obtain 100-1000 demonstrations of a new undertaking or robot, employing a robotic arm managed by a human.
- High-quality-tune RoboCat on this new task/arm, developing a specialised spin-off agent.
- The spin-off agent practises on this new undertaking/arm an common of 10,000 instances, making a lot more training facts.
- Include the demonstration data and self-created information into RoboCat’s existing training dataset.
- Train a new edition of RoboCat on the new coaching dataset.
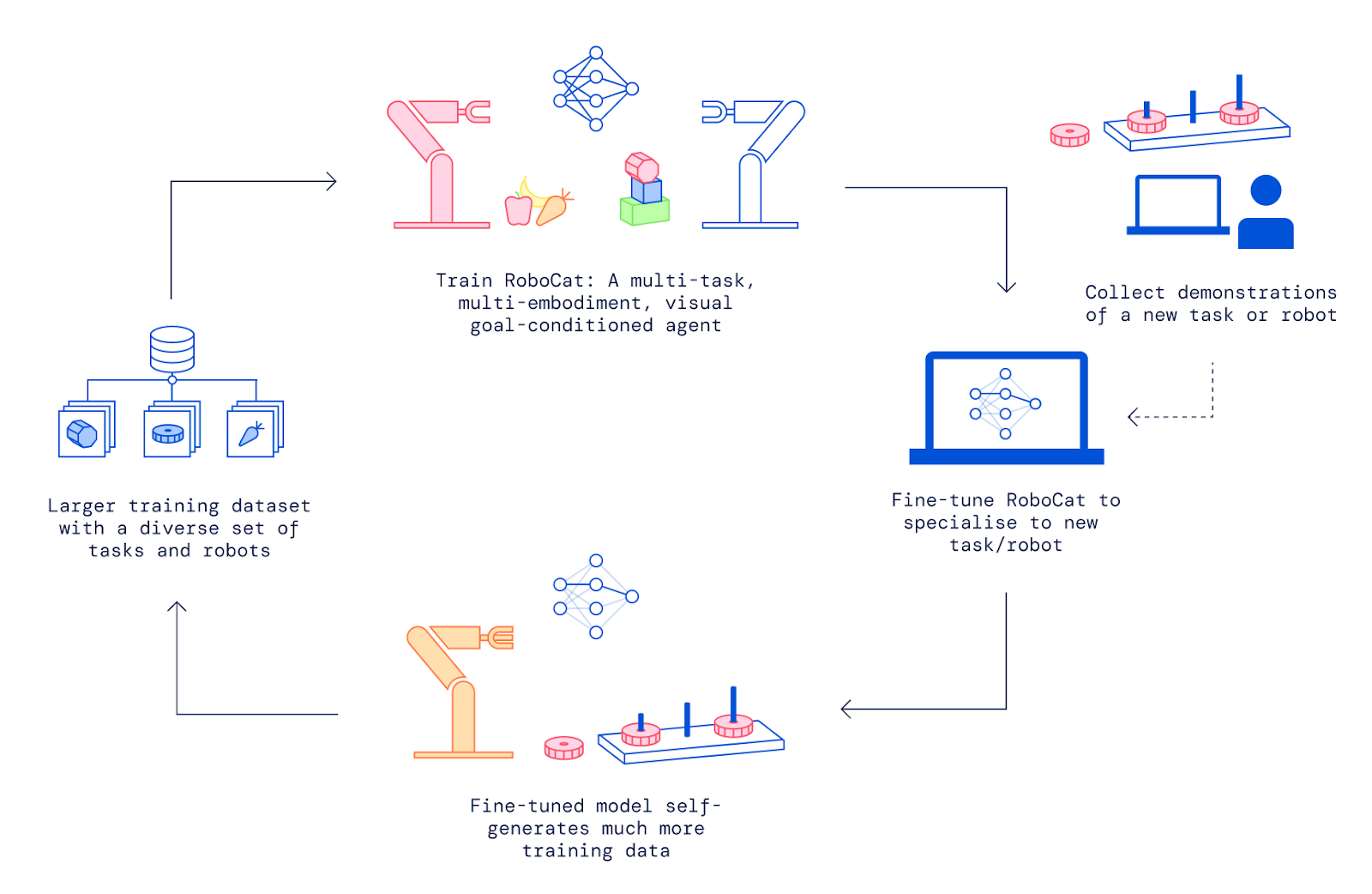
The mixture of all this coaching suggests the most up-to-date RoboCat is dependent on a dataset of tens of millions of trajectories, from both genuine and simulated robotic arms, which includes self-produced information. We utilised four different styles of robots and a lot of robotic arms to gather vision-primarily based data symbolizing the duties RoboCat would be skilled to perform.
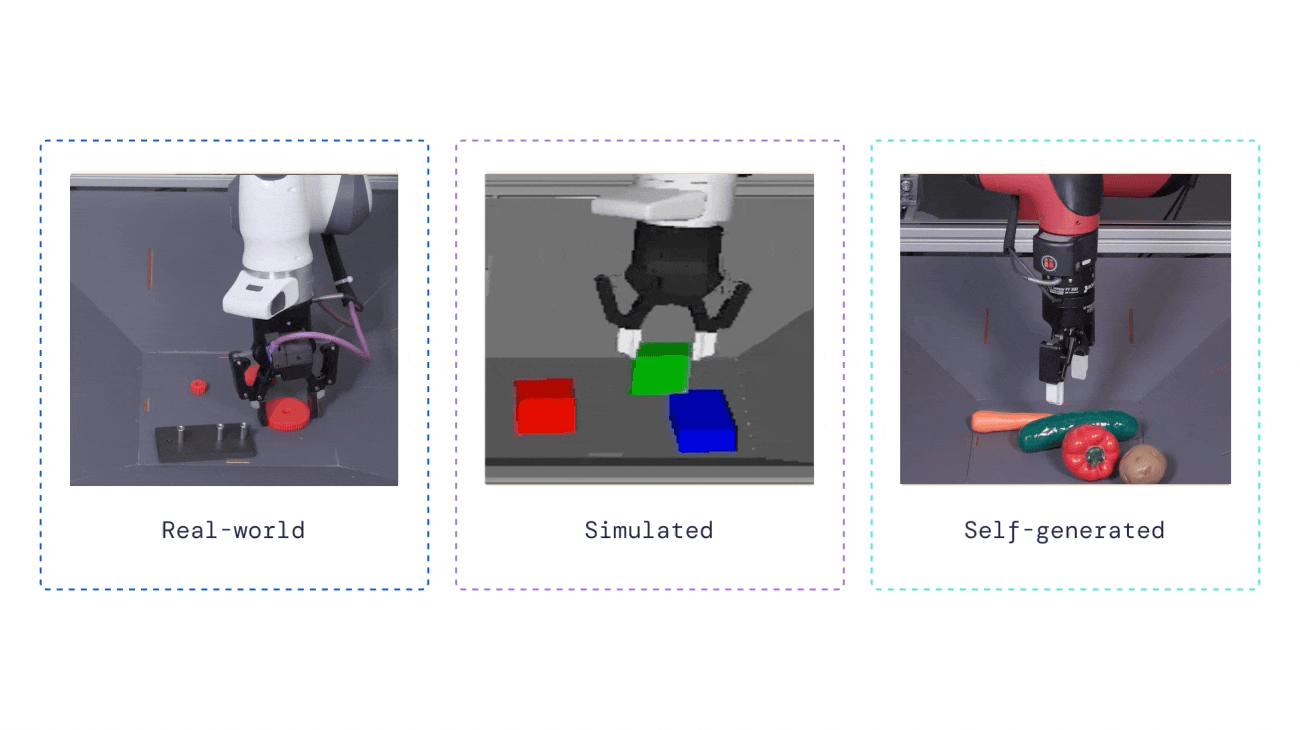
Learning to operate new robotic arms and resolve more complex jobs
With RoboCat’s diverse schooling, it figured out to operate distinct robotic arms in just a couple hours. While it had been trained on arms with two-pronged grippers, it was ready to adapt to a a lot more complex arm with a a few-fingered gripper and twice as many controllable inputs.
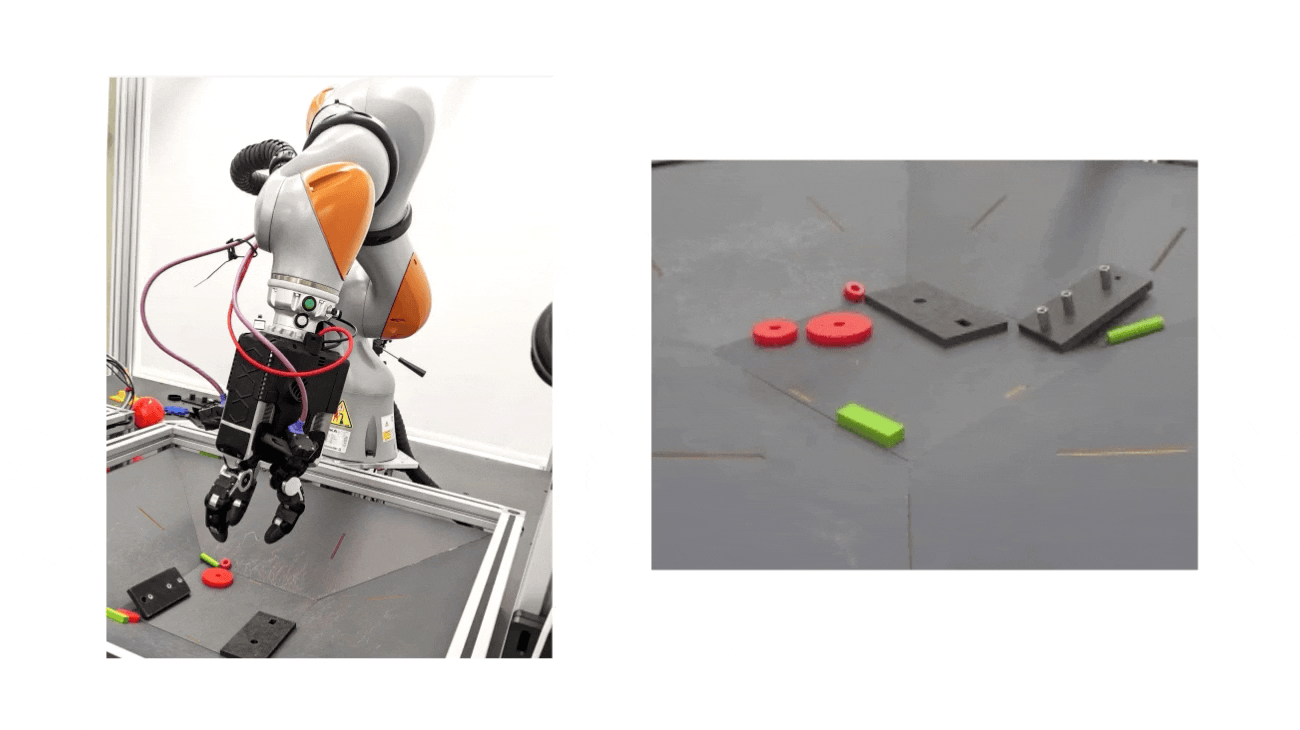
Ideal: Video of RoboCat utilizing the arm to decide on up gears
Soon after observing 1000 human-controlled demonstrations, gathered in just hrs, RoboCat could direct this new arm dexterously plenty of to pick up gears properly 86% of the time. With the very same stage of demonstrations, it could adapt to clear up duties that merged precision and comprehension, this sort of as removing the accurate fruit from a bowl and solving a form-matching puzzle, which are necessary for more elaborate regulate.
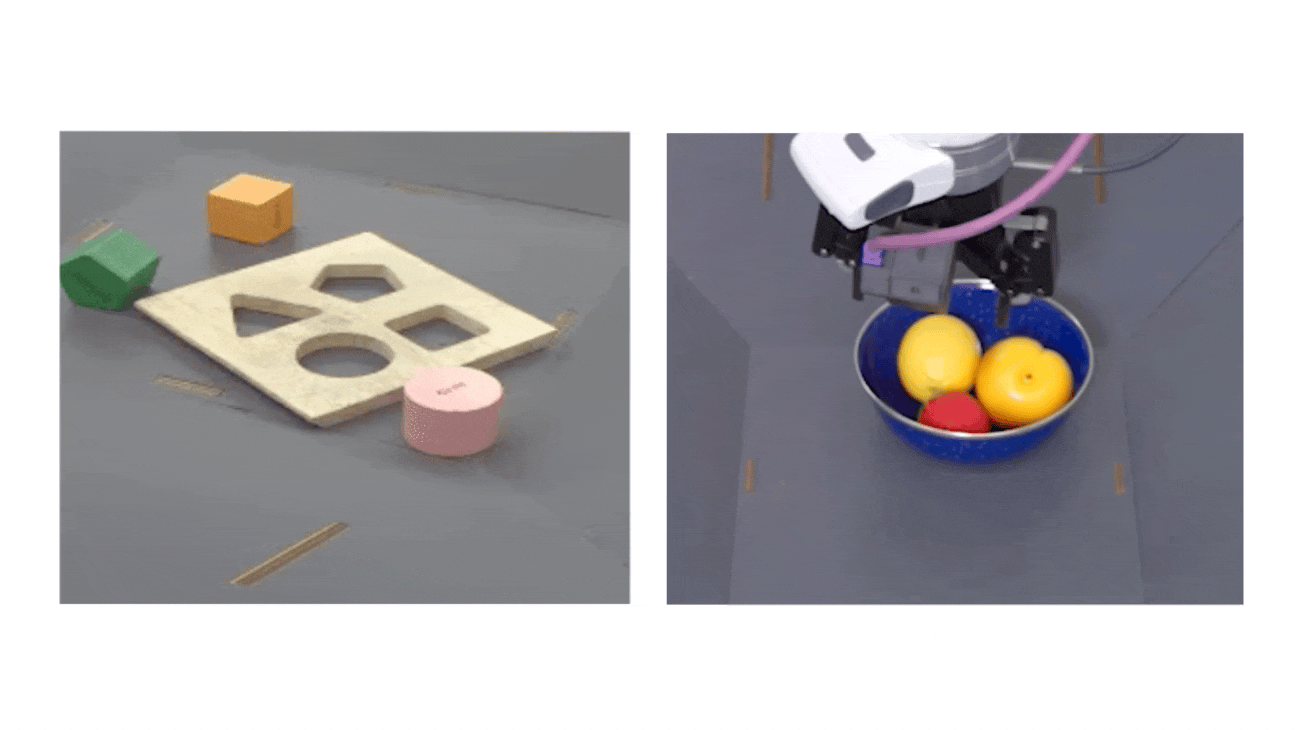
The self-improving generalist
RoboCat has a virtuous cycle of schooling: the far more new tasks it learns, the better it will get at understanding further new tasks. The initial model of RoboCat was effective just 36% of the time on formerly unseen jobs, soon after discovering from 500 demonstrations for each undertaking. But the most recent RoboCat, which had trained on a better variety of duties, more than doubled this accomplishment price on the very same responsibilities.
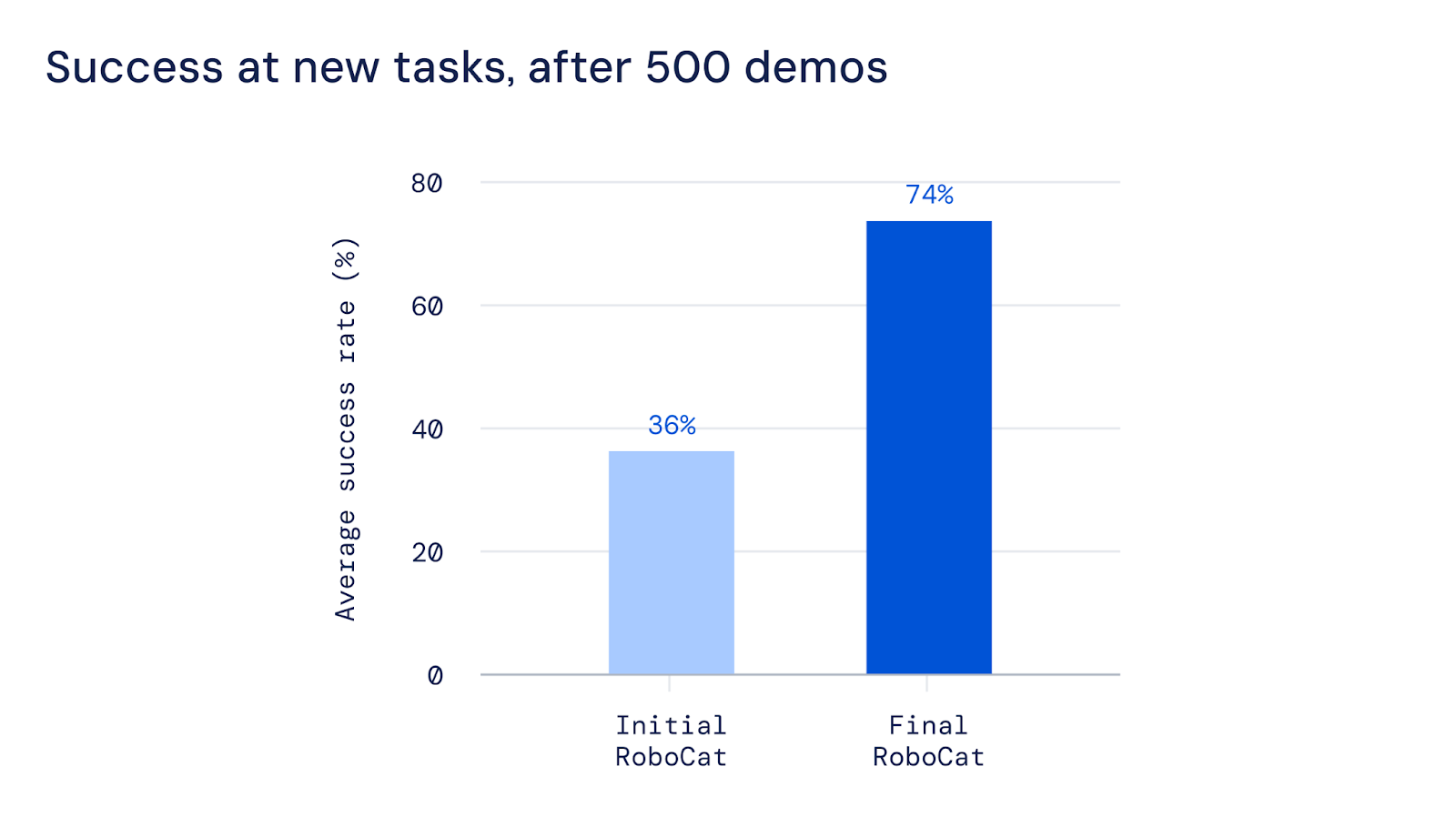
These advancements were being because of to RoboCat’s developing breadth of working experience, equivalent to how people develop a far more assorted variety of techniques as they deepen their finding out in a specified domain. RoboCat’s means to independently discover competencies and speedily self-make improvements to, especially when utilized to unique robotic products, will aid pave the way towards a new technology of more beneficial, common-objective robotic brokers.
[ad_2]
Supply website link