[ad_1]
Resolving some of the major difficulties of the 21st Century, such as producing clear electrical energy or creating superior temperature superconductors, will require us to style and design new materials with unique qualities. To do this on a laptop or computer demands the simulation of electrons, the subatomic particles that govern how atoms bond to form molecules and are also responsible for the stream of electric power in solids. Even with many years of effort and numerous considerable innovations, accurately modelling the quantum mechanical conduct of electrons continues to be an open up problem. Now, in a paper (Open up Obtain PDF) revealed in Science, we propose DM21, a neural network attaining point out of the artwork accuracy on huge sections of chemistry. To speed up scientific development, we’re also open up sourcing our code for anybody to use.
Practically a century in the past, Erwin Schrödinger proposed his famous equation governing the behaviour of quantum mechanical particles. Applying this equation to electrons in molecules is challenging for the reason that all electrons repel just about every other. This would seem to be to require monitoring the likelihood of each electron’s posture — a remarkably sophisticated task for even a modest range of electrons. A single major breakthrough came in the 1960s, when Pierre Hohenberg and Walter Kohn realised that it is not needed to keep track of every electron separately. Instead, understanding the chance for any electron to be at every single placement (i.e., the electron density) is adequate to specifically compute all interactions. Kohn gained a Nobel Prize in Chemistry after proving this, consequently founding Density Purposeful Theory (DFT).
Although DFT proves a mapping exists, for more than 50 yrs the exact character of this mapping in between electron density and interaction power — the so-known as density useful — has remained not known and has to be approximated. In spite of the point that DFT intrinsically will involve a level of approximation, it is the only functional technique to review how and why make any difference behaves in a sure way at the microscopic level and has thus develop into 1 of the most broadly utilised techniques in all of science. Around the yrs, researchers have proposed many approximations to the exact practical with different amounts of accuracy. Even with their recognition, all of these approximations put up with from systematic glitches because they are unsuccessful to seize selected critical mathematical houses of the correct practical.
By expressing the useful as a neural community and incorporating these precise qualities into the education knowledge, we understand functionals totally free from important systematic mistakes — resulting in a far better description of a broad class of chemical reactions.
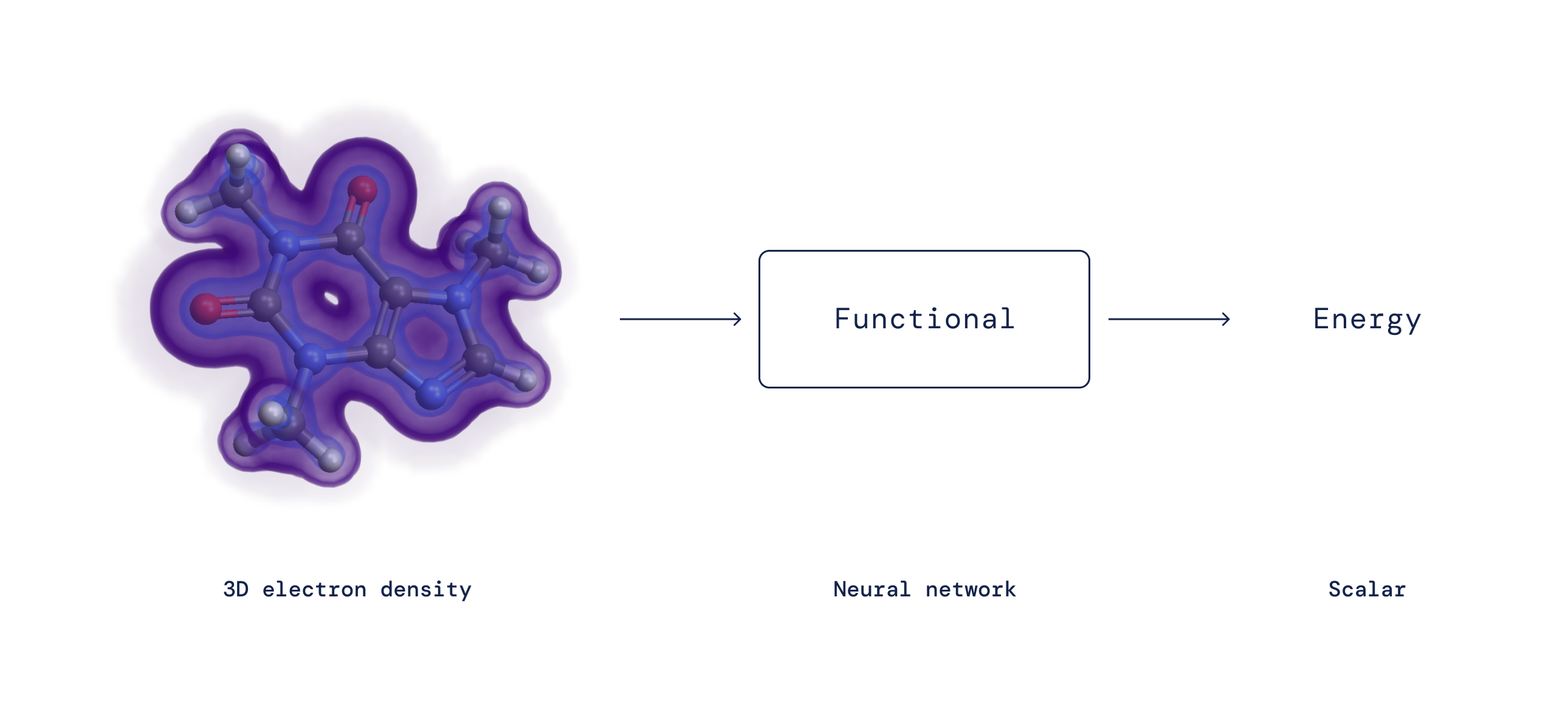
We precisely tackle two lengthy-standing complications with classic functionals:
- The delocalization error: In a DFT calculation, the functional establishes the demand density of a molecule by discovering the configuration of electrons which minimizes vitality. Consequently, problems in the practical can guide to faults in the calculated electron density. Most present density functional approximations prefer electron densities that are unrealistically unfold out above a number of atoms or molecules instead than being effectively localized around a single molecule or atom (see Fig 2).
- Spin symmetry breaking: When describing the breaking of chemical bonds, present functionals tend to unrealistically favor configurations in which a essential symmetry recognized as spin symmetry is damaged. Considering that symmetries participate in a critical purpose in our understanding of physics and chemistry, this synthetic symmetry breaking reveals a significant deficiency in current functionals.
In basic principle, any chemical-bodily process that will involve movement of demand is liable to suffer from delocalization error, and any process that will involve the breaking of bonds is liable to suffer from spin-symmetry breaking. Motion of charge and bond breaking are core to many significant technological purposes, but these troubles can also guide to large qualitative failure of functionals to explain the simplest molecules, these kinds of as hydrogen. Considering that DFT is this kind of a critical technology it is critical to style and design functionals that get this basic chemistry correct ahead of inquiring them to reveal vastly more elaborate molecular interactions, this sort of as those that may possibly take place in a battery or solar cell.
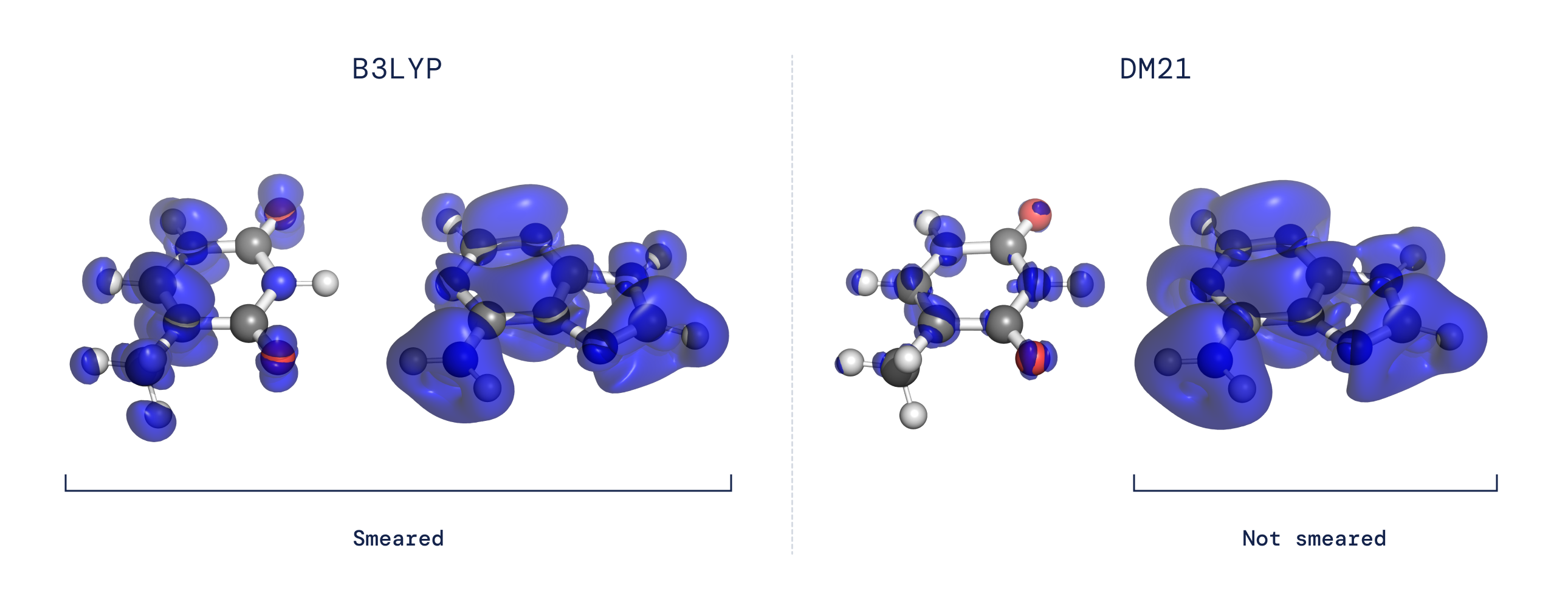
These longstanding difficulties are both of those related to how functionals behave when offered with a process that reveals “fractional electron character.” By employing a neural network to signify the practical and tailoring our training dataset to capture the fractional electron conduct anticipated for the precise purposeful, we located that we could solve the difficulties of delocalization and spin symmetry-breaking. Our functional also showed by itself to be hugely exact on broad, substantial-scale benchmarks, suggesting that this data-pushed approach can seize areas of the specific functional that have therefore considerably been elusive.
For years, laptop or computer simulations have performed a central purpose in contemporary engineering, making it attainable to deliver reliable responses to concerns like “will this bridge keep up?” to “will this rocket make it into place?” As technological know-how increasingly turns to the quantum scale to check out questions about products, medicines, and catalysts, which includes all those we’ve under no circumstances found or even imagined, deep understanding demonstrates guarantee to properly simulate issue at this quantum mechanical amount.
[ad_2]
Resource backlink