[ad_1]
In our latest paper we explore how multi-agent deep reinforcement finding out can provide as a product of elaborate social interactions, like the formation of social norms. This new course of styles could deliver a route to make richer, extra specific simulations of the entire world.
Humans are an ultra social species. Relative to other mammals we advantage extra from cooperation but we are also extra dependent on it, and facial area greater cooperation troubles. These days, humanity faces quite a few cooperation troubles including avoiding conflict about resources, ensuring absolutely everyone can access clean up air and drinking h2o, removing extreme poverty, and combating local climate adjust. Numerous of the cooperation difficulties we face are challenging to take care of because they require sophisticated webs of social and biophysical interactions called social-ecological devices. Nonetheless, human beings can collectively study to overcome the cooperation challenges we encounter. We accomplish this by an ever evolving lifestyle, which include norms and institutions which manage our interactions with the atmosphere and with a person an additional.
Even so, norms and institutions at times fail to resolve cooperation worries. For example, individuals may possibly more than-exploit means like forests and fisheries thus producing them to collapse. In these kinds of scenarios, coverage-makers may perhaps create guidelines to improve institutional policies or produce other interventions to try out to alter norms in hopes of bringing about a favourable improve. But coverage interventions do not generally operate as meant. This is simply because true-entire world social-ecological devices are considerably additional advanced than the types we normally use to try out to forecast the effects of applicant policies.
Designs primarily based on sport concept are typically applied to the review of cultural evolution. In most of these designs, the vital interactions that brokers have with a single another are expressed in a ‘payoff matrix’. In a activity with two contributors and two actions A and B, a payoff matrix defines the worth of the 4 doable outcomes: (1) we equally decide on A, (2) we equally opt for B, (3) I decide on A although you opt for B and (4) I decide on B whilst you pick A. The most well-known example is the ‘Prisoner’s Dilemma’, in which the steps are interpreted as “cooperate” and “defect”. Rational agents who act according to their possess myopic self-fascination are doomed to defect in the Prisoner’s Predicament even although the superior result of mutual cooperation is readily available.
Activity-theoretic designs have been really extensively used. Researchers in diverse fields have used them to research a huge array of diverse phenomena, like economies and the evolution of human lifestyle. Nonetheless, activity principle is not a neutral tool, fairly it is a deeply opinionated modeling language. It imposes a demanding necessity that everything have to finally hard cash out in conditions of the payoff matrix (or equal representation). This means that the modeler has to know, or be inclined to presume, all the things about how the outcomes of individual steps merge to produce incentives. This is sometimes acceptable, and the recreation theoretic tactic has experienced many notable successes this sort of as in modeling the habits of oligopolistic companies and cold war era intercontinental relations. Even so, recreation theory’s significant weak spot as a modeling language is uncovered in scenarios in which the modeler does not entirely realize how the selections of folks blend to make payoffs. Sad to say this tends to be the situation with social-ecological units mainly because their social and ecological elements interact in complex techniques that we do not entirely have an understanding of.
The get the job done we existing listed here is a single instance inside of a investigate plan that attempts to build an alternative modeling framework, diverse from activity principle, to use in the analyze of social-ecological devices. Our solution may possibly be viewed formally as a selection of agent-primarily based modeling. Having said that, its distinguishing function is the incorporation of algorithmic components from artificial intelligence, especially multi-agent deep reinforcement understanding.
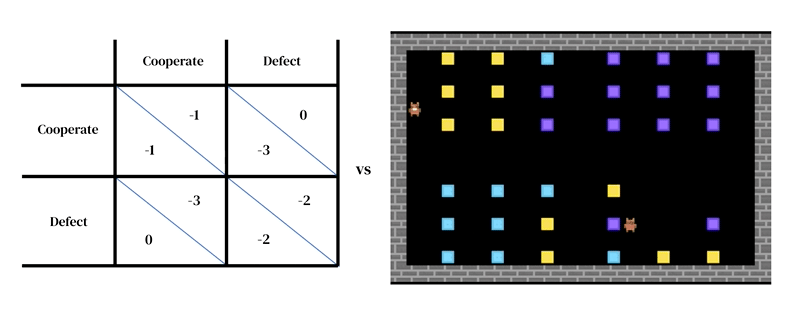
The core notion of this approach is that each design consists of two interlocking parts: (1) a abundant, dynamical product of the atmosphere and (2) a model of person choice-generating.
The initially requires the variety of a researcher-designed simulator: an interactive system that usually takes in a present-day natural environment condition and agent steps, and outputs the upcoming ecosystem condition as perfectly as the observations of all agents and their instantaneous rewards. The product of specific decision-earning is likewise conditioned on ecosystem point out. It is an agent that learns from its earlier knowledge, carrying out a variety of demo-and-error. An agent interacts with an environment by taking in observations and outputting steps. Just about every agent selects steps in accordance to its behavioral plan, a mapping from observations to actions. Brokers discover by switching their policy to make improvements to it together any preferred dimension, usually to attain extra reward. The plan is saved in a neural network. Agents study ‘from scratch’, from their individual working experience, how the planet is effective and what they can do to receive more benefits. They attain this by tuning their network weights in these a way that the pixels they acquire as observations are slowly remodeled into skilled actions. Numerous finding out brokers can inhabit the very same natural environment as 1 a further. In this circumstance the agents turn into interdependent mainly because their steps impact one particular an additional.
Like other agent-dependent modeling approaches, multi-agent deep reinforcement learning tends to make it simple to specify products that cross degrees of analysis that would be challenging to handle with recreation theory. For instance, steps may well be significantly closer to very low-stage motor primitives (e.g. ‘walk forward’ ‘turn right’) than the high-amount strategic conclusions of game theory (e.g. ‘cooperate’). This is an critical aspect essential to seize scenarios the place agents will have to exercise to discover correctly how to implement their strategic decisions. For instance in 1 study, agents learned to cooperate by getting turns cleansing a river. This answer was only achievable since the surroundings had spatial and temporal proportions in which agents have good flexibility in how they composition their habits to a single one more. Interestingly, although the environment authorized for several various remedies (this sort of as territoriality), brokers converged on the exact same change-having answer as human gamers.
In our most recent research, we used this style of product to an open up issue in analysis on cultural evolution: how to demonstrate the existence of spurious and arbitrary social norms that show up not to have immediate materials outcomes for their violation past people imposed socially. For instance, in some societies adult men are predicted to use trousers not skirts in a lot of there are words or hand gestures that ought to not be utilised in well mannered organization and in most there are policies about how a person models one’s hair or what a person wears on one’s head. We connect with these social norms ‘silly rules’. Importantly, in our framework, imposing and complying with social norms each have to be acquired. Having a social natural environment that contains a ‘silly rule’ signifies that brokers have additional prospects to master about implementing norms in normal. This added practice then enables them to enforce the vital guidelines additional effectively. All round, the ‘silly rule’ can be effective for the populace – a shocking result. This final result is only attainable since our simulation focuses on finding out: enforcing and complying with principles are complicated capabilities that have to have schooling to establish.
Part of why we obtain this consequence on silly procedures so remarkable is that it demonstrates the utility of multi-agent deep reinforcement finding out in modeling cultural evolution. Society contributes to the success or failure of coverage interventions for socio-ecological programs. For instance, strengthening social norms all around recycling is element of the solution to some environmental challenges. Following this trajectory, richer simulations could direct to a further comprehension of how to style and design interventions for social-ecological programs. If simulations turn into realistic enough, it may even be probable to check the affect of interventions, e.g. aiming to style and design a tax code that fosters productivity and fairness.
This method supplies researchers with tools to specify in depth types of phenomena that curiosity them. Of program, like all analysis methodologies it should be expected to occur with its very own strengths and weaknesses. We hope to explore a lot more about when this design and style of modeling can be fruitfully utilized in the long run. Though there are no panaceas for modeling, we assume there are persuasive motives to search to multi-agent deep reinforcement mastering when constructing types of social phenomena, especially when they entail finding out.
[ad_2]
Source website link